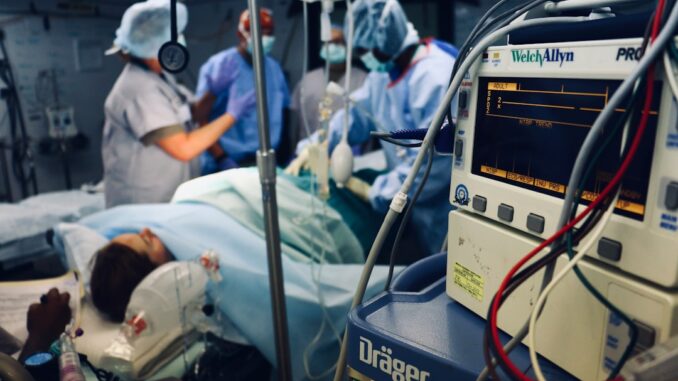
Summary
A new AI model accurately predicts blood transfusion needs in non-traumatic ICU patients, potentially improving patient outcomes and resource allocation. This breakthrough utilizes machine learning and a vast dataset of patient records to identify key biomarkers and predict transfusion requirements within a 24-hour window. This technology has the power to transform critical care medicine by enhancing decision-making and optimizing blood product utilization.
Healthcare data growth can be overwhelming scale effortlessly with TrueNAS by Esdebe.
Main Story
Artificial intelligence is, no doubt, shaking things up in healthcare, and there’s this new application making some real noise in the ICU. You might find this interesting; researchers over at Emory University have cooked up an AI model that can actually predict if a non-trauma ICU patient is going to need a blood transfusion. It’s a pretty big deal for critical care medicine. Think about it: this could mean better outcomes for patients, smart use of blood supplies, and way faster decision-making in the high-stress environment of the ICU.
This study, which was published in Health Data Science, tackles a problem that’s been around for ages. How do you accurately predict who’s going to need a transfusion? It’s not easy, especially when dealing with all sorts of patients who have varying conditions. Most systems out there are pretty limited, they only focus on specific groups or types of transfusions. This new model is different. It dives into a whole range of clinical data, from lab results to vital signs, and then predicts transfusion needs within a 24-hour period. It’s pretty impressive.
Developing this AI wasn’t a walk in the park, though. The Emory team used a massive dataset–over 72,000 ICU patient records collected over five years. Can you imagine wrangling that much data? It’s what you need though. Collected from a large metropolitan hospital, this dataset allowed them to really train and validate the model. By using machine learning and a ‘meta-model ensemble’ approach – a bit technical, I know! – they got some amazing results. The AI has an AUROC of 0.97, an accuracy rate of 0.93, and an F1 score of 0.89. In layman’s terms, that means it’s super reliable and has very strong predictive power.
What’s particularly interesting, is how the model zeroes in on key biomarkers. It’s not just about predicting; it helps clinicians understand why a patient might need a transfusion, focusing on things like hemoglobin and platelet levels. This means treatments can be much more tailored to each patient’s needs, with clinicians having advanced warning of potential problems. And as a result, the patient might have an easier time. That’s the hope anyway!
It doesn’t just improve prediction. It also optimizes blood product use. Blood shortages are an ongoing problem in many hospitals, and making sure that resources are used effectively is really important; especially in the ICU, where every second counts. I remember a time at my old hospital during flu season, the blood bank was under so much pressure it was crazy. This model could help with those kinds of situations by streamlining processes and enhancing clinical decision-making. It’s a huge deal when it comes to making ICU care more efficient.
Next up, the research team is working on getting this AI model into clinical workflows for real-time support. This’ll let them see how effective it is in actual ICU settings. The real goal here is to get a tool available that allows clinicians to provide the very best care for patients. For me, this study really highlights the transformative potential of AI, how it can address complex challenges and help improve patient outcomes.
That said, let’s be clear, this AI isn’t going to replace clinicians, it is a tool to help them do a better job. Human oversight and medical judgment are still critical. Besides, more research is needed to refine the model. That needs to happen so it can be used for even more patients and situations. But really, the future for AI in healthcare is promising, and this blood transfusion model is a great example of how much things are changing. It’s pretty cool, isn’t it?
The meta-model ensemble approach sounds fascinating. How might this technique be applied to other diagnostic or predictive healthcare models beyond blood transfusions?
That’s a great question! The meta-model ensemble’s ability to integrate diverse data points is definitely powerful. I think it could be very useful in other areas like predicting sepsis or assessing risk for cardiovascular events where a range of factors need to be considered.
Editor: MedTechNews.Uk
Thank you to our Sponsor Esdebe – https://esdebe.com
72,000 records? I bet the data wranglers needed a blood transfusion after that effort! Hopefully, the AI can predict their needs next time.
Haha, you’re absolutely right! The data wrangling was definitely a huge undertaking, I can only imagine. It really does highlight the value in creating tools to predict not just patient needs, but potentially even resource demands for research too!
Editor: MedTechNews.Uk
Thank you to our Sponsor Esdebe – https://esdebe.com
So, this AI can predict transfusions, but can it predict when the coffee machine in the ICU will break down? That’s a real emergency, surely?
That’s a great point! Predicting critical equipment failures, like coffee machines or ventilators, could be another valuable application of AI in the ICU, ensuring smooth operations and reducing stress on the medical teams as well as the patients!
Editor: MedTechNews.Uk
Thank you to our Sponsor Esdebe – https://esdebe.com
The focus on key biomarkers is particularly insightful, potentially leading to more personalized transfusion strategies beyond just predicting the need.
Thanks for highlighting that point! The potential for personalized strategies is definitely an exciting aspect. The AI’s ability to identify key biomarkers could allow clinicians to fine-tune their transfusion approaches for better patient outcomes.
Editor: MedTechNews.Uk
Thank you to our Sponsor Esdebe – https://esdebe.com