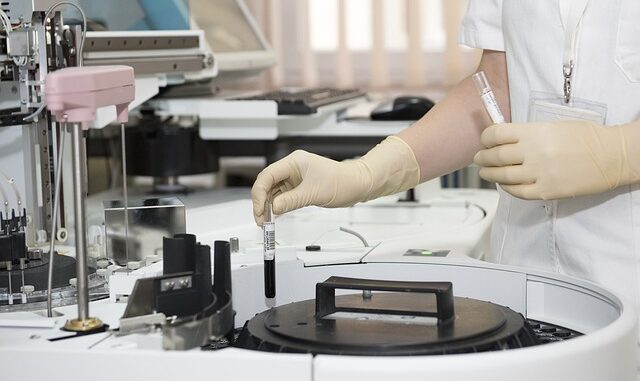
Summary
This article explores the crucial role of digital infrastructure in scaling AI-powered screening solutions within the healthcare sector. It examines the challenges and opportunities associated with data interoperability, cybersecurity, and strategic data integration. By combining robust frameworks with effective data management, healthcare organizations can unlock the full potential of AI for early disease detection and improved patient outcomes.
** Main Story**
Alright, let’s talk about scaling AI in medical screening. It’s a hot topic, and for good reason. I mean, the FDA’s already given the thumbs up to over 1,000 healthcare algorithms, many geared towards early disease detection. It’s exciting, right? But here’s the thing: all that AI power is useless without the right foundation.
We need a serious, solid digital infrastructure to truly make AI work in healthcare. It’s not just about having the algorithms, it’s about making sure they can actually do their job effectively. Think of it like having a race car but no track to drive on.
The Data Dilemma: Interoperability is Key
One of the biggest hurdles? Data, data everywhere, but not a drop to drink. Okay, maybe not that bad, but you get the idea. Healthcare data is so scattered, isn’t it? It lives in different systems that don’t always talk to each other. That lack of interoperability – the ability for those systems to actually exchange information – well, it’s a huge problem. Without it, we can’t get a complete picture of a patient’s medical history. And if AI can’t access all the relevant information, its predictions won’t be as accurate as they could be. Consider this, Okan Ekinci, a cardiologist over at Roche, puts it well. He reckons that “Data fluidity is becoming so important because we need to make sure AI-based medical algorithms can access the right data for all patients at the right time.”
Now, I remember a few years ago, working on a project where we were trying to implement a machine learning model to predict hospital readmissions. We spent months just wrangling the data, trying to get it into a format the model could understand. Seriously, that was a learning curve. So getting that data flowing seamlessly, is key to unlocking the true potential of personalized, precision-based care. And isn’t that the goal?
Don’t Forget Cybersecurity (Because Nobody Wants a Data Breach)
Let’s be real; when we’re talking about integrating AI into healthcare IT systems, cybersecurity isn’t an optional extra, it’s absolutely fundamental. Patient data is incredibly sensitive, and we’re obliged to keep it protected. HIPAA, for example, is not something you want to mess with. These algorithms, they often need access to huge amounts of data to work their magic, which means we’ve got to build a secure digital ecosystem from the ground up. It is crucial for maintaining patient trust, and the law!
Strategic Data Integration and Governance? Absolutely!
So, you’ve got the tech, great, but AI success also needs strategic thinking and solid governance. I mean, it’s not just about throwing an algorithm at a problem and hoping for the best. You have to really understand your patient population – their demographics, their health needs. Why? Because that’s how you tailor AI solutions to actually be effective.
And speaking of being effective, analyzing demographic data? It’s a powerful way to show just how beneficial AI screening tools can be for public health. It helps make the case for adoption, you know? Plus, a strong governance process, involving leaders from across different departments, is really important for overseeing the design and implementation of those algorithms. No one wants an algorithm that reinforces biases or makes inaccurate diagnoses.
Building Blocks for AI Greatness (or, How to Not Have Your System Crash)
Alright, what does this “solid digital infrastructure” even look like? Well, there are some key components to consider:
- High-Density Colocation: Think data centers built to handle insane amounts of data. This is how AI applications can run smoothly. Which brings us to…
- Robust Capacity: We’re talking high-performance power and cooling systems because these AI workloads are intense. You don’t want your system to overheat, right?
- Sustainability: Environmentally friendly energy and cooling methods. It’s good for the planet and can save you money in the long run. It’s also important to have regulatory compliance, because you might as well get ahead of the curve.
- Strategic Partnerships: Teaming up with industry leaders to stay on top of the latest AI advancements. You can’t do it all alone. It’s worth remembering that you will need collaboration and working together with other vendors and solutions.
By putting all of that in place, organizations can really unlock the full potential of AI, leading to sustainable growth, efficiency, and agility in their healthcare operations. Now that’s something everyone can get behind.
Don’t Forget the Humans!
Let’s face it, we can get lost in the tech, but never forget, people are important in healthcare. Technology forms the spine of AI, the human element is just as crucial, if not more so. So healthcare professionals must be involved in the development and oversight of these algorithms. Ekinci underlines this point, stressing how people are needed in the process. AI is meant to augment clinical judgement, not replace it, after all. That ensures AI tools lead to more informed and effective healthcare decisions. It’s about collaboration, finding the sweet spot where human expertise and technological innovation meet. That is how we are going to improve patient outcomes.
So, we’re trusting AI to detect diseases early, but relying on digital infrastructure that sounds like it was designed in the Stone Age? High-density colocation sounds fancy, but is anyone addressing the inherent biases in the *algorithms* themselves, or are we just scaling flawed logic faster?
That’s a great point about the algorithms themselves! While robust infrastructure is key, addressing biases within the AI is crucial. Algorithmic fairness and ethical considerations are definitely areas that need more focus as we scale these technologies. Thanks for bringing this up!
Editor: MedTechNews.Uk
Thank you to our Sponsor Esdebe
So, we’re trusting AI to detect diseases early, but relying on digital infrastructure that sounds like it was designed in the Stone Age? High-density colocation sounds fancy, but is anyone addressing the inherent biases in the *algorithms* themselves, or are we just scaling flawed logic faster?
That’s a really important question! It’s not just about scaling, it’s about *how* we scale. Ensuring fairness means constant vigilance, diverse datasets, and ongoing algorithm audits. The ‘Stone Age’ infrastructure you mentioned is improving, but the algorithmic bias issue requires even more urgent attention and collaboration. How do you think regulation can assist?
Editor: MedTechNews.Uk
Thank you to our Sponsor Esdebe