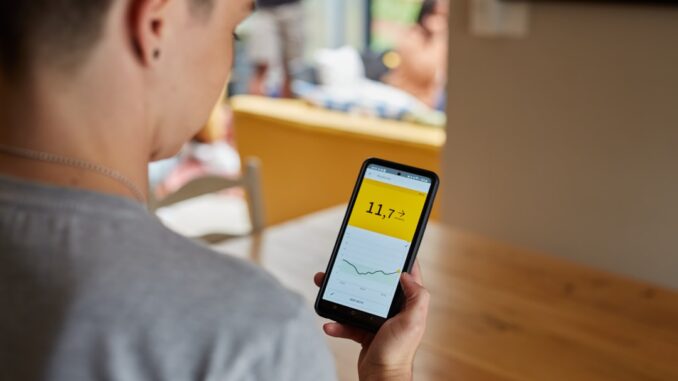
Summary
Continuous glucose monitors (CGMs) are revolutionizing diabetes care, as new research demonstrates their ability to predict diabetes-related complications like nerve, eye, and kidney damage. This breakthrough allows for earlier intervention and personalized treatment strategies, potentially preventing life-altering complications. The study found that time spent in a safe blood-sugar range, as measured by CGMs, is as effective as traditional hemoglobin A1c levels in predicting complications.
Safeguard patient information with TrueNASs self-healing data technology.
Main Story
Okay, let’s talk about diabetes management, it’s going through a real revolution right now, thanks to continuous glucose monitoring, or CGMs. I mean, it’s pretty wild how much things have changed. Turns out, these little devices aren’t just tracking your sugar in real time, they’re also capable of predicting some nasty complications like nerve, eye, and kidney damage. Seriously, that’s huge!
For years, you know, we’ve relied on A1c tests, which gives you an average blood sugar over a couple of months. It’s like looking at a blurry photo, it’s ok, but doesn’t really show you the whole picture. While A1c is alright, it totally misses the crazy swings your blood sugar can take during the day. CGMs, though? They give you a minute-by-minute play of what’s happening. It’s like having a real time video feed rather than just a snapshot.
Now, some smart people at the University of Virginia took a look at old data from a massive study, they called it the DCCT, from the 90’s. It was a pivotal study. They used some fancy machine learning tricks to create virtual CGM readings from that old data. Pretty clever, right? Then they compared the CGM info against the old school A1c results. The question was, which one is better for predicting the risk of those nasty complications?
The findings? Well, it’s very interesting. The time someone spent with their glucose in a target range, around 70 to 180 mg/dL, over just 14 days as recorded by the virtual CGM, was just as good at predicting neuropathy, retinopathy, and nephropathy as the A1c. That’s just wild! So, CGM isn’t just a fancy toy, it’s a game-changer.
And that’s not all! The study also discovered that how much time your blood sugar spent above certain high levels is also a good indicator of future issues. This is important because it emphasizes the point of keeping those spikes down and your levels nice and stable, you know?
Think about it, with CGMs, doctors can now see who is at high risk early, and personalize their care. This means tweaking meds, pushing for lifestyle changes, or upping the monitoring. The earlier you get on top of it, the better, it’s so crucial for avoiding or slowing down these complications. You might ask, “How much better can it really be?” Well, it’s a big step.
That said, CGMs are also incredibly empowering for people with diabetes. It’s like having your own little glucose coach, telling you if your sugar is too high or too low, right there on your phone. For example, I know someone who, after getting her CGM, was able to fine-tune her meals so her blood sugar would stop going crazy after lunch. Its a massive deal and patients can really take a hands on approach with their care.
So while the A1c is still useful, CGMs offer a complimentary, and more detailed view of your blood sugar. It’s like having a high definition picture versus one that is grainy. In other words, it’s a major leap for diabetes care, and could help a lot of people live healthier lives. Frankly, I’m pretty excited about the future of diabetes research and all the new ways we’ll use this cool tech to make lives better. Who knows what other amazing things we’ll discover!
The reliance on machine learning to generate virtual CGM data from a 1990s study introduces significant limitations. The translation of this data into current clinical practice requires further validation and consideration of advancements in diabetes management since that time.
That’s a very valid point about the limitations of using machine learning with older data. It does highlight the need for further research. We definitely need to validate these findings with more contemporary data sets and consider how advancements in diabetes management have shifted our understanding of optimal care.
Editor: MedTechNews.Uk
Thank you to our Sponsor Esdebe – https://esdebe.com
“Fancy machine learning tricks” to create virtual data from the 90s? Sounds like someone was playing Sim Diabetes. I bet you can buy NFTs of those data points.
That’s a funny comparison! It’s amazing how machine learning can extrapolate insights from older data, almost like creating a time machine for our glucose levels. It does open up a lot of questions about how to use old and new data.
Editor: MedTechNews.Uk
Thank you to our Sponsor Esdebe – https://esdebe.com
So, the 90’s are back in fashion, and now diabetes data too? Next thing you know, everyone will be rocking digital beepers showing their glucose trends.
That’s a fun image! It’s interesting how revisiting older datasets with new technology can uncover fresh perspectives, like comparing a 90’s snapshot with today’s continuous monitoring. It really highlights how far data analysis has come, wouldn’t you agree?
Editor: MedTechNews.Uk
Thank you to our Sponsor Esdebe – https://esdebe.com
So, the 90s data is like a vintage synth sample being remixed for modern diabetes management. Next they’ll be finding lost floppy disks with even older glucose readings.
That’s a great analogy! The remixing concept really highlights how we can learn from older data with new tech. Imagine the possibilities if we could unearth more data insights from the past!
Editor: MedTechNews.Uk
Thank you to our Sponsor Esdebe – https://esdebe.com
The study’s reliance on virtual CGM data from the 90s, despite machine learning, remains questionable. The predictive power of this data needs substantial validation before impacting current clinical protocols.
That’s a fair point about validation. The study’s findings are interesting, but it’s crucial to confirm the predictive power of the virtual CGM data against more contemporary, real-world data before any change in clinical practice. It opens a great discussion about the balance between innovative analysis and reliable clinical application.
Editor: MedTechNews.Uk
Thank you to our Sponsor Esdebe – https://esdebe.com
The study’s finding that time in range correlates with complication risk, as well as A1c, offers a new metric for evaluating treatment effectiveness. This could inform more personalized patient care plans.
Absolutely! It’s exciting to see how time in range can offer a more granular view than A1c alone. This opens avenues for tailoring treatment plans more effectively to individual needs and glucose patterns.
Editor: MedTechNews.Uk
Thank you to our Sponsor Esdebe – https://esdebe.com
The study’s focus on time in range as a predictor of complications, equivalent to A1c, demonstrates the value of dynamic glucose data. This warrants exploring how this metric might be incorporated into treatment guidelines.
I agree completely. The potential for incorporating time in range into treatment guidelines is very exciting. It really opens up possibilities for more proactive and personalized care plans, moving beyond the limitations of a single A1c number.
Editor: MedTechNews.Uk
Thank you to our Sponsor Esdebe – https://esdebe.com