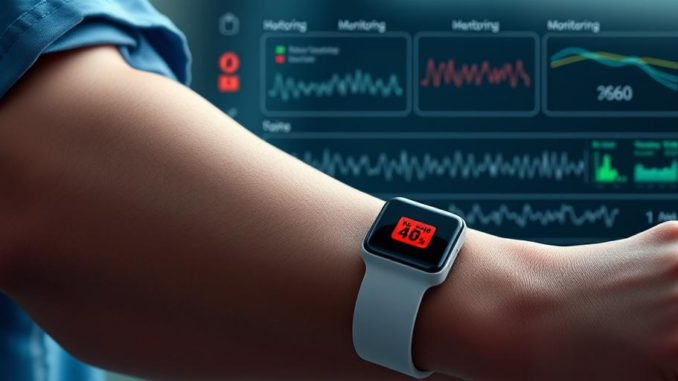
Advanced Hemodynamic Monitoring: From Invasive Techniques to Wearable Technologies and Future Directions
Abstract
Hemodynamic monitoring is a cornerstone of modern critical care and cardiovascular medicine, providing crucial insights into the circulatory system’s function and guiding therapeutic interventions. This report explores the evolution of hemodynamic monitoring, from traditional invasive methods to the emerging field of wearable, non-invasive technologies. We delve into the clinical significance of key hemodynamic parameters, evaluate the strengths and limitations of both invasive and non-invasive techniques, and examine the potential applications of wearable monitoring in diverse patient populations. Furthermore, we discuss the algorithmic foundations that underpin the interpretation of data from wearable devices, addressing challenges related to data accuracy, validation, and clinical integration. Finally, we explore the future directions of hemodynamic monitoring, highlighting areas ripe for innovation and potential advancements that could revolutionize patient care.
1. Introduction
The circulatory system’s efficiency in delivering oxygen and nutrients to tissues is paramount for maintaining cellular function and overall health. Hemodynamic monitoring provides a window into this complex system, allowing clinicians to assess cardiac output, blood pressure, oxygen delivery, and other critical parameters. These measurements guide decisions regarding fluid management, vasopressor administration, and other therapies aimed at optimizing circulatory function. Historically, hemodynamic monitoring relied heavily on invasive techniques, requiring the placement of catheters into major blood vessels. While these methods offer detailed and accurate data, they are associated with potential complications and are not suitable for continuous, long-term monitoring in ambulatory settings. The advent of non-invasive techniques has broadened the scope of hemodynamic monitoring, enabling more frequent and less risky assessments. Recently, wearable technologies have emerged as a promising avenue for continuous, non-invasive monitoring, offering the potential to transform the management of cardiovascular diseases.
2. Clinical Significance of Hemodynamic Parameters
A comprehensive understanding of hemodynamic parameters is crucial for interpreting monitoring data and making informed clinical decisions. Key parameters include:
-
Heart Rate (HR): The number of heartbeats per minute, reflecting the heart’s activity. Tachycardia (elevated HR) can indicate dehydration, pain, or underlying cardiac pathology, while bradycardia (slow HR) may suggest medication effects, conduction abnormalities, or underlying metabolic disorders.
-
Blood Pressure (BP): The force exerted by blood against the arterial walls, encompassing systolic BP (SBP) during ventricular contraction and diastolic BP (DBP) during ventricular relaxation. Hypotension (low BP) can result in inadequate tissue perfusion, leading to organ damage. Hypertension (high BP) increases the risk of cardiovascular events, such as stroke and myocardial infarction.
-
Oxygen Saturation (SpO2): The percentage of hemoglobin saturated with oxygen, reflecting the adequacy of oxygen delivery to tissues. Hypoxemia (low SpO2) can result from pulmonary disease, cardiac dysfunction, or impaired oxygen transport.
-
Cardiac Output (CO): The volume of blood pumped by the heart per minute, reflecting the heart’s pumping efficiency. CO is determined by stroke volume (SV), the volume of blood ejected with each heartbeat, and heart rate. Low CO can lead to inadequate tissue perfusion and shock.
-
Stroke Volume (SV): The volume of blood ejected by the left ventricle during each contraction. It is a key determinant of cardiac output. SV is influenced by preload (the volume of blood filling the ventricles), afterload (the resistance the heart must overcome to eject blood), and contractility (the force of ventricular contraction).
-
Systemic Vascular Resistance (SVR): The resistance to blood flow in the systemic circulation. High SVR increases the heart’s workload, while low SVR can contribute to hypotension.
-
Central Venous Pressure (CVP): The pressure in the superior vena cava, reflecting right atrial pressure and serving as an estimate of right ventricular preload. CVP monitoring can guide fluid management in critically ill patients. However, it’s recognized that CVP is not always a reliable predictor of fluid responsiveness.
Understanding the interplay between these parameters is essential for diagnosing and managing a wide range of cardiovascular conditions. For example, in a patient with heart failure, monitoring CO, SV, and SVR can help guide the selection of appropriate medications to improve cardiac function and reduce afterload.
3. Traditional Methods of Hemodynamic Monitoring
Traditional hemodynamic monitoring techniques are broadly classified into invasive and non-invasive methods.
3.1 Invasive Hemodynamic Monitoring
Invasive methods involve the placement of catheters into blood vessels or the heart to directly measure hemodynamic parameters. The gold standard for invasive monitoring is the pulmonary artery catheter (PAC), also known as the Swan-Ganz catheter. The PAC allows for the measurement of pulmonary artery pressure (PAP), pulmonary capillary wedge pressure (PCWP), CVP, and CO. PCWP provides an estimate of left atrial pressure and left ventricular preload. However, the use of PACs has declined in recent years due to concerns about complications and a lack of evidence demonstrating improved outcomes in all patient populations [1].
Arterial lines are commonly used to continuously monitor blood pressure and obtain arterial blood samples for blood gas analysis. They are typically placed in the radial, femoral, or brachial artery. While arterial lines provide accurate BP measurements, they are associated with risks, including infection, thrombosis, and bleeding.
Esophageal Doppler monitoring (EDM) is a minimally invasive technique that uses a Doppler probe placed in the esophagus to measure aortic blood flow velocity. EDM can provide estimates of CO and SV. However, its accuracy can be affected by probe placement and patient positioning.
3.2 Non-Invasive Hemodynamic Monitoring
Non-invasive methods do not require the insertion of catheters into blood vessels or the heart. These techniques are safer and more convenient than invasive methods, making them suitable for a wider range of patients and settings.
Non-invasive blood pressure (NIBP) measurement is routinely performed using oscillometric devices. These devices inflate a cuff around the arm or leg and measure BP based on the oscillations in cuff pressure. While NIBP is convenient, it can be less accurate than invasive BP monitoring, particularly in patients with hypotension or arrhythmias.
Echocardiography uses ultrasound to visualize the heart and assess its structure and function. Echocardiography can provide estimates of CO, SV, and ejection fraction (EF), the percentage of blood ejected from the left ventricle with each contraction. Echocardiography is a valuable tool for diagnosing heart failure, valvular disease, and other cardiac conditions. Transesophageal echocardiography (TEE) offers improved image quality compared to transthoracic echocardiography (TTE) but is a semi-invasive procedure.
Bioimpedance and bioreactance technologies measure changes in electrical impedance or radiofrequency signals across the thorax to estimate CO. These techniques are non-invasive and relatively easy to use, but their accuracy can be affected by patient factors, such as fluid status and body composition.
Pulse contour analysis (PCA) is a technique that estimates CO and SV based on the shape of the arterial pressure waveform. PCA can be performed using both invasive and non-invasive arterial pressure monitoring systems. The accuracy of PCA can be affected by changes in arterial compliance and vascular tone.
4. Continuous and Non-Invasive Monitoring: Advantages and Limitations
The ability to continuously monitor hemodynamic parameters non-invasively offers several advantages over intermittent measurements and invasive techniques.
4.1 Advantages
-
Early Detection of Hemodynamic Instability: Continuous monitoring allows for the early detection of subtle changes in hemodynamic parameters that may precede overt clinical deterioration. This can enable timely interventions to prevent adverse outcomes.
-
Personalized Fluid Management: Continuous monitoring can guide fluid administration in patients at risk for fluid overload or dehydration. By tracking changes in CO, SV, and CVP, clinicians can optimize fluid balance and improve tissue perfusion.
-
Improved Titration of Vasoactive Medications: Continuous monitoring facilitates the precise titration of vasopressors and inotropes to maintain adequate BP and CO. This can minimize the risk of adverse effects associated with excessive or inadequate medication doses.
-
Reduced Risk of Complications: Non-invasive monitoring eliminates the risks associated with invasive procedures, such as infection, bleeding, and thrombosis.
-
Enhanced Patient Comfort and Mobility: Non-invasive monitoring is generally more comfortable for patients than invasive monitoring, allowing for greater mobility and participation in rehabilitation activities.
-
Feasibility in Ambulatory Settings: Non-invasive monitoring can be used in ambulatory settings to monitor patients with chronic cardiovascular conditions, such as heart failure and hypertension.
4.2 Limitations
Despite these advantages, continuous and non-invasive monitoring techniques also have limitations:
-
Accuracy Concerns: Non-invasive methods may be less accurate than invasive methods, particularly in patients with complex medical conditions or significant hemodynamic instability. The accuracy of non-invasive techniques can be affected by patient factors, such as obesity, arrhythmias, and peripheral vascular disease.
-
Data Interpretation Challenges: Interpreting continuous hemodynamic data requires expertise and experience. Clinicians must be able to differentiate between clinically significant changes and artifacts or noise.
-
Limited Parameter Coverage: Some non-invasive techniques provide only limited information about hemodynamic parameters. For example, NIBP only measures BP and does not provide information about CO or SV.
-
Algorithm Dependence: The accuracy of many non-invasive hemodynamic monitors depends on the algorithms used to analyze the data. These algorithms may not be accurate in all patient populations or clinical situations.
-
Integration into Clinical Workflows: Integrating continuous hemodynamic data into clinical workflows can be challenging. Clinicians must be able to access and interpret the data in a timely and efficient manner.
5. Wearable Hemodynamic Monitoring: Applications and Challenges
Wearable hemodynamic monitoring devices, such as smartwatches and adhesive patches, represent a significant advancement in the field. These devices offer the potential for continuous, non-invasive monitoring in ambulatory settings, enabling remote patient management and early detection of cardiovascular events.
5.1 Potential Applications
-
Chronic Heart Failure Management: Wearable monitoring can track hemodynamic parameters, such as HR, BP, and SpO2, in patients with chronic heart failure. This information can be used to detect early signs of decompensation and guide medication adjustments to prevent hospitalizations.
-
Hypertension Management: Wearable devices can continuously monitor BP in patients with hypertension, providing valuable data for medication titration and lifestyle modifications. Ambulatory BP monitoring can also help identify white-coat hypertension and masked hypertension.
-
Diabetes Management: Patients with diabetes are at increased risk for cardiovascular disease. Wearable monitoring can track hemodynamic parameters and detect early signs of cardiac dysfunction.
-
Post-Operative Monitoring: Wearable devices can be used to monitor patients after surgery, detecting early signs of complications, such as bleeding, infection, and arrhythmias.
-
Athletic Performance Monitoring: Wearable devices can track HR, BP, and SpO2 in athletes, providing insights into their cardiovascular fitness and helping them optimize their training regimens.
-
Sleep Apnea Screening: Some wearable devices can detect changes in SpO2 during sleep, which may indicate sleep apnea.
5.2 Algorithms Used in Wearable Hemodynamic Monitoring
The accuracy and reliability of wearable hemodynamic monitoring devices depend heavily on the algorithms used to interpret the sensor data. These algorithms typically employ a combination of signal processing techniques, machine learning, and physiological models.
-
Heart Rate Estimation: Heart rate is often estimated from the photoplethysmography (PPG) signal, which measures changes in blood volume in the microvasculature. Algorithms analyze the PPG signal to identify the peaks corresponding to each heartbeat. These algorithms often incorporate filtering techniques to remove noise and artifacts.
-
Blood Pressure Estimation: Blood pressure estimation is more challenging than heart rate estimation. Some wearable devices use oscillometric methods to measure BP, similar to traditional NIBP devices. Others use pulse transit time (PTT), the time it takes for a pulse wave to travel between two arterial sites, as a surrogate for BP. PTT is inversely correlated with BP. Algorithms that use PTT typically require calibration against a reference BP measurement.
-
Oxygen Saturation Estimation: Oxygen saturation is estimated from the ratio of red and infrared light absorption in the PPG signal. Algorithms use calibration curves to convert the ratio of light absorption to SpO2 values.
-
Motion Artifact Reduction: Motion artifacts are a major challenge in wearable hemodynamic monitoring. Algorithms employ various techniques to reduce motion artifacts, such as adaptive filtering, wavelet transforms, and accelerometer-based motion compensation. These methods attempt to separate the physiological signal from the noise caused by movement.
-
Machine Learning Approaches: Machine learning algorithms, such as neural networks and support vector machines, are increasingly being used to improve the accuracy of hemodynamic parameter estimation. These algorithms can learn complex relationships between sensor data and hemodynamic parameters, potentially leading to more accurate and robust measurements.
5.3 Challenges and Limitations
Despite the potential benefits of wearable hemodynamic monitoring, several challenges and limitations must be addressed before these devices can be widely adopted in clinical practice:
-
Data Accuracy and Validation: The accuracy of wearable hemodynamic monitoring devices can be affected by various factors, including sensor placement, skin tone, motion artifacts, and environmental conditions. Rigorous validation studies are needed to assess the accuracy and reliability of these devices in diverse patient populations.
-
Algorithm Robustness: The algorithms used in wearable devices must be robust to variations in patient physiology and environmental conditions. Algorithms should be trained and tested on large datasets to ensure their generalizability.
-
Data Interpretation and Clinical Integration: Clinicians need to be trained on how to interpret data from wearable hemodynamic monitoring devices and integrate it into clinical decision-making. Guidelines and protocols are needed to standardize the use of these devices in clinical practice.
-
Data Security and Privacy: Wearable devices collect sensitive patient data, which must be protected from unauthorized access. Robust security measures are needed to ensure data privacy and confidentiality.
-
Battery Life and User Compliance: Wearable devices must have sufficient battery life to support continuous monitoring. They should also be comfortable and easy to use to promote user compliance.
-
Regulatory Approval: Wearable hemodynamic monitoring devices that are intended for medical use must undergo regulatory review and approval by agencies such as the FDA.
-
Lack of Standardized Metrics and Protocols: There is a lack of standardized metrics and protocols for evaluating the performance of wearable hemodynamic monitoring devices. This makes it difficult to compare the performance of different devices and to assess their clinical utility.
6. Future Directions
The field of hemodynamic monitoring is rapidly evolving, with ongoing research focused on developing more accurate, reliable, and user-friendly technologies. Future directions include:
-
Advanced Sensor Technologies: Development of new sensor technologies that are less susceptible to motion artifacts and environmental noise. Examples include implantable sensors, optical sensors that penetrate deeper into the tissue, and sensors that measure multiple hemodynamic parameters simultaneously.
-
Artificial Intelligence and Machine Learning: Leveraging AI and machine learning to improve the accuracy of hemodynamic parameter estimation, predict adverse events, and personalize treatment strategies. Specifically, the development of predictive models that integrate hemodynamic data with other clinical information to identify patients at high risk for decompensation.
-
Integration with Electronic Health Records (EHRs): Seamless integration of data from wearable devices into EHRs to facilitate clinical decision-making and improve patient care coordination. Addressing interoperability challenges to enable data sharing across different healthcare systems.
-
Telemedicine and Remote Patient Monitoring: Expanding the use of wearable hemodynamic monitoring in telemedicine and remote patient monitoring programs to improve access to care and reduce healthcare costs.
-
Closed-Loop Control Systems: Development of closed-loop control systems that automatically adjust medication dosages based on continuous hemodynamic monitoring data. For example, closed-loop systems could automatically titrate vasopressors to maintain target BP levels.
-
Personalized Hemodynamic Profiles: Creating personalized hemodynamic profiles for each patient based on their individual physiological characteristics and disease state. This could lead to more tailored and effective treatment strategies.
-
Improved Validation Methodologies: Developing more rigorous validation methodologies for wearable hemodynamic monitoring devices. This includes the use of standardized protocols, larger sample sizes, and diverse patient populations.
-
Addressing Ethical Considerations: Addressing ethical considerations related to data privacy, security, and the potential for misuse of wearable hemodynamic monitoring data.
7. Conclusion
Hemodynamic monitoring has undergone a remarkable transformation, from invasive techniques used primarily in critical care settings to wearable technologies that enable continuous, non-invasive monitoring in ambulatory settings. While traditional invasive methods remain essential for critically ill patients, non-invasive and wearable technologies offer the potential to improve the management of cardiovascular diseases in a broader range of patients. However, challenges related to data accuracy, algorithm robustness, and clinical integration must be addressed before these technologies can be widely adopted in clinical practice. Continued research and development in sensor technologies, artificial intelligence, and data analytics will drive further advancements in hemodynamic monitoring, ultimately leading to improved patient outcomes and reduced healthcare costs.
References
[1] Wheeler, D. S., Devarajan, P., & Moffett, B. S. (2013). Pulmonary artery catheter use in pediatric critical care: a survey of practice patterns. Pediatric Critical Care Medicine, 14(7), 692-698.
[2] Marik, P. E., Baram, M., & Vahid, B. (2008). Does central venous pressure predict fluid responsiveness? A systematic review of the literature and the tale of seven mares. Chest, 134(1), 172-178.
[3] Cecconi, M., De Backer, D., Antonelli, M., Beale, R., Bakker, J., Hofer, C., … & Payen, D. (2014). Consensus on circulatory shock and hemodynamic monitoring. Task force of the European Society of Intensive Care Medicine. Intensive Care Medicine, 40(12), 1795-1815.
[4] Mukkamala, R., Stergiou, G. S., Bramos, D., Patzak, A., Wassertheurer, S., Avolio, A. P., … & Sharman, J. E. (2018). Method for obtaining blood pressure measurements using a smartphone. Scientific Reports, 8(1), 1-10.
[5] Allen, J. (2007). Photoplethysmography and its application in clinical physiological measurement. Physiological Measurement, 28(3), R1.
[6] Elgendi, M. (2012). On the analysis of fingertip photoplethysmogram signals. Current Cardiology Reviews, 8(1), 14-25.
[7] Poon, C. C. Y., & Zhang, Y. T. (2006). Cuffless blood pressure estimation from pulse transit time using a support vector machine. IEEE Transactions on Biomedical Engineering, 53(12), 2682-2694.
[8] Ramkumar, P., Mukkamala, R., & Natarajan, K. (2020). Towards cuffless blood pressure estimation with a chest-worn wearable sensor. IEEE Transactions on Biomedical Engineering, 67(10), 2831-2841.
[9] Slapnicar, I., Mlakar, N., Luštrek, M., & Matjačić, Z. (2019). Evaluation of motion artifact reduction techniques for photoplethysmography-based heart rate estimation during physical exercise. Sensors, 19(17), 3842.
[10] Ding, X. R., Wu, Y., Chen, W., Yang, G. Z., & Huang, H. (2016). Deriving respiratory rate from pulse oximetry by means of frequency domain analysis. Journal of Medical Engineering & Technology, 40(7-8), 399-407.
The discussion of integrating AI and machine learning to improve hemodynamic parameter estimation is particularly compelling. Could these advancements lead to predictive models capable of anticipating patient decompensation before traditional indicators manifest?
That’s a great point! The potential for AI to predict decompensation is a game-changer. Imagine using machine learning to analyze subtle hemodynamic shifts, allowing for earlier intervention and improved patient outcomes. It opens doors to proactive, personalized care strategies. What are your thoughts on the ethical implications of predictive AI in healthcare?
Editor: MedTechNews.Uk
Thank you to our Sponsor Esdebe
This is an insightful overview. The discussion of motion artifact reduction in wearable monitoring is crucial. How can we best leverage sensor fusion, combining data from multiple sensors like accelerometers and gyroscopes, to enhance signal processing and improve the reliability of hemodynamic measurements during real-world activities?
Thank you for highlighting the importance of motion artifact reduction! Sensor fusion is definitely key. Combining accelerometer and gyroscope data with advanced signal processing techniques like Kalman filtering could significantly improve the accuracy and robustness of wearable hemodynamic monitoring, especially during dynamic activities. Great point!
Editor: MedTechNews.Uk
Thank you to our Sponsor Esdebe
Wearable tech predicting heart failure? Sounds like a sci-fi movie, but with better actors (hopefully!). Wonder if we’ll soon be diagnosing ourselves with algorithms from our wrist? Asking for a friend… who is also a hypochondriac.
Haha, love the sci-fi comparison! It’s true, the thought of wrist-worn diagnostics can feel a bit futuristic. But, imagine the possibilities for early detection and preventative care! Maybe the hypochondriac ‘friend’ is just ahead of the curve? Thanks for the fun take on it!
Editor: MedTechNews.Uk
Thank you to our Sponsor Esdebe
The section on personalized hemodynamic profiles is intriguing. The ability to tailor treatment strategies based on individual physiological characteristics could significantly improve patient outcomes, particularly in managing complex cardiovascular conditions. What are the key challenges in gathering and interpreting the data needed to create these profiles?