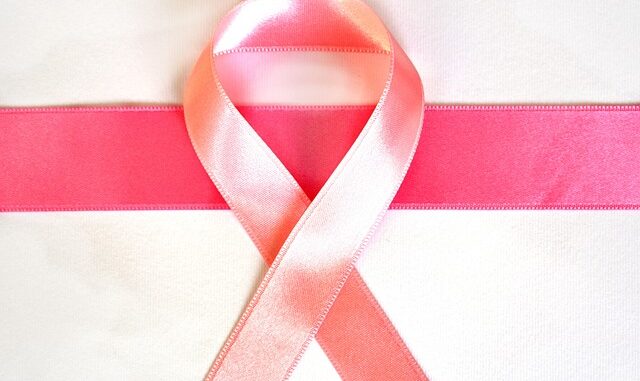
Advances in Early Cancer Detection: A Multi-Modal and Emerging Technology Perspective
Many thanks to our sponsor Esdebe who helped us prepare this research report.
Abstract
Early cancer detection is paramount for improving patient outcomes. This report provides a comprehensive overview of current and emerging technologies in this crucial field. It examines conventional methods like imaging, liquid biopsies, and computational pathology, highlighting their strengths and limitations. Furthermore, the report explores the potential of innovative technologies such as artificial intelligence, nanotechnology, and quantum sensors in revolutionizing early cancer diagnosis. The integration of multi-modal approaches, combining different diagnostic techniques, is also discussed, emphasizing the synergy in achieving higher sensitivity and specificity. The challenges associated with translating these advancements into clinical practice, including regulatory hurdles, cost-effectiveness, and ethical considerations, are also addressed. Finally, the report concludes with a discussion on future research directions and the potential for personalized cancer screening strategies.
Many thanks to our sponsor Esdebe who helped us prepare this research report.
1. Introduction
Cancer remains a leading cause of mortality worldwide, with survival rates strongly correlated with the stage at diagnosis [1]. Early detection enables timely intervention, improving the chances of successful treatment and long-term survival [2]. However, many cancers are asymptomatic in their early stages, leading to delayed diagnosis and poorer prognosis. Therefore, the development and implementation of effective early cancer detection strategies are of paramount importance.
This report aims to provide a comprehensive overview of the current state-of-the-art in early cancer detection, encompassing both established and emerging technologies. We will explore the limitations of current methodologies and delve into the potential of novel approaches to overcome these challenges. The integration of multi-modal strategies, combining different diagnostic modalities, will be highlighted as a crucial approach to enhancing the accuracy and reliability of early cancer detection. Finally, we will discuss the challenges and future directions in this rapidly evolving field.
Many thanks to our sponsor Esdebe who helped us prepare this research report.
2. Conventional Cancer Detection Methods
2.1. Imaging Techniques
Imaging plays a central role in cancer detection, staging, and monitoring. Conventional imaging modalities include:
- X-ray: While widely available and relatively inexpensive, X-ray imaging has limited sensitivity for detecting small tumors and involves exposure to ionizing radiation [3]. Mammography, a specific application of X-ray imaging, is a standard screening tool for breast cancer, but its sensitivity can be reduced in women with dense breast tissue [4].
- Computed Tomography (CT): CT scans provide detailed cross-sectional images of the body, enabling the detection of tumors in various organs. However, CT scans also involve ionizing radiation and may require the use of contrast agents, which can cause adverse reactions in some patients [5].
- Magnetic Resonance Imaging (MRI): MRI offers excellent soft tissue contrast without the use of ionizing radiation. It is particularly useful for imaging the brain, spinal cord, and soft tissues. However, MRI is more expensive than CT and may not be suitable for patients with certain metallic implants [6].
- Ultrasound: Ultrasound is a non-invasive and relatively inexpensive imaging technique that uses sound waves to create images of internal organs. It is commonly used for imaging the liver, gallbladder, and kidneys. However, ultrasound images can be affected by factors such as body habitus and the presence of gas in the bowel [7].
- Positron Emission Tomography (PET): PET scans use radioactive tracers to detect areas of increased metabolic activity, which can indicate the presence of cancer. PET scans are often combined with CT scans (PET/CT) to provide both anatomical and functional information [8].
The limitations of these imaging techniques include their reliance on detecting relatively large tumors, their exposure to ionizing radiation (in some cases), and their potential for false-positive and false-negative results. There is also a drive toward lower-dose radiation protocols where possible.
2.2. Liquid Biopsies
Liquid biopsies involve the analysis of biological fluids, such as blood, for the presence of cancer-related biomarkers. This approach offers several advantages over traditional tissue biopsies, including its non-invasiveness, ease of repeatability, and ability to capture tumor heterogeneity [9]. Key biomarkers analyzed in liquid biopsies include:
- Circulating Tumor Cells (CTCs): CTCs are cancer cells that have detached from the primary tumor and are circulating in the bloodstream. The enumeration and characterization of CTCs can provide valuable information about disease progression and treatment response [10]. However, CTCs are often rare in the circulation, making their detection challenging.
- Circulating Tumor DNA (ctDNA): ctDNA consists of DNA fragments released into the bloodstream by tumor cells. The analysis of ctDNA can identify cancer-specific mutations and track changes in tumor burden [11]. ctDNA is generally more abundant than CTCs, making its detection more feasible. However, ctDNA can also be present in healthy individuals due to clonal hematopoiesis of indeterminate potential (CHIP), which can lead to false-positive results [12].
- Exosomes: Exosomes are small vesicles secreted by cells that contain a variety of molecules, including proteins, RNA, and DNA. Cancer cells release exosomes that can promote tumor growth and metastasis. The analysis of exosomal cargo can provide insights into tumor biology and identify potential therapeutic targets [13].
While liquid biopsies hold great promise for early cancer detection, several challenges remain, including the standardization of assays, the interpretation of results, and the cost-effectiveness of implementation.
2.3. Computational Pathology
Computational pathology leverages image analysis, machine learning, and artificial intelligence to extract quantitative information from digitized histopathology slides [14]. This approach can improve the accuracy and efficiency of cancer diagnosis by identifying subtle features that may be missed by the human eye. Computational pathology can also be used to predict prognosis and treatment response based on histopathological features [15]. The algorithms can be trained to identify specific cell types, quantify tumor heterogeneity, and assess the expression of biomarkers. However, the development and validation of computational pathology algorithms require large and well-annotated datasets. There is also the issue of algorithm bias and generalisability across different patient populations and laboratory settings [16].
Many thanks to our sponsor Esdebe who helped us prepare this research report.
3. Emerging Technologies for Early Cancer Detection
3.1. Artificial Intelligence (AI)
AI, particularly machine learning and deep learning, is transforming cancer detection across various modalities. AI algorithms can be trained to analyze medical images, liquid biopsy data, and histopathology slides to identify patterns indicative of cancer [17]. For example, AI can improve the accuracy of mammography screening by reducing false-positive rates and detecting subtle lesions that may be missed by radiologists [18]. AI can also be used to predict the risk of cancer based on clinical and genomic data [19]. The development of robust and reliable AI algorithms requires large and diverse datasets and careful validation to ensure that they generalize well to different patient populations. There are also concerns about the interpretability of AI algorithms, which can make it difficult to understand why they make certain predictions. However, explainable AI (XAI) is an active area of research to address this concern [20].
3.2. Nanotechnology
Nanotechnology offers the potential to develop highly sensitive and specific cancer detection tools. Nanoparticles can be engineered to target cancer cells and deliver contrast agents for imaging or therapeutic agents for treatment [21]. Nanoparticles can also be used to capture and concentrate cancer-related biomarkers from liquid biopsies, improving the sensitivity of detection [22]. For example, gold nanoparticles can be functionalized with antibodies that bind to CTCs, allowing for their capture and enumeration [23]. Quantum dots, which are fluorescent semiconductor nanocrystals, can be used to label cancer cells and track their movement in vivo [24]. The development of nanotechnology-based cancer detection tools requires careful consideration of their biocompatibility and potential toxicity. The long-term effects of nanoparticles on human health are still not fully understood, and rigorous safety testing is required before they can be used in clinical practice.
3.3. Quantum Sensors
Quantum sensors are highly sensitive devices that can detect subtle changes in physical properties, such as magnetic fields, temperature, and pressure. These sensors have the potential to revolutionize cancer detection by detecting early signs of cancer that are not detectable by conventional methods [25]. For example, nitrogen-vacancy (NV) centers in diamond can be used to detect magnetic fields generated by cancer cells [26]. Quantum sensors can also be used to measure the metabolic activity of cancer cells, providing insights into their growth and behavior [27]. The development of quantum sensors for cancer detection is still in its early stages, but the potential benefits are significant. Challenges include the miniaturization of quantum sensors, the development of biocompatible materials, and the integration of quantum sensors with existing diagnostic platforms.
3.4. Multi-Omics Approaches
Multi-omics approaches integrate data from different “omics” domains, such as genomics, transcriptomics, proteomics, and metabolomics, to provide a comprehensive view of cancer biology [28]. By analyzing multiple layers of biological information, multi-omics approaches can identify biomarkers that are more sensitive and specific than those identified by single-omics approaches. For example, the integration of genomic and proteomic data can identify cancer subtypes that are associated with different clinical outcomes [29]. Multi-omics approaches can also be used to predict treatment response and identify potential drug targets [30]. The analysis of multi-omics data requires sophisticated bioinformatics tools and statistical methods. The interpretation of multi-omics data can be challenging due to the complexity of biological systems. However, the potential benefits of multi-omics approaches for early cancer detection and personalized medicine are significant.
Many thanks to our sponsor Esdebe who helped us prepare this research report.
4. Multi-Modal Cancer Detection
Combining multiple diagnostic modalities, known as multi-modal cancer detection, has the potential to significantly improve the accuracy and reliability of early cancer diagnosis [31]. By integrating information from different sources, such as imaging, liquid biopsies, and genomics, multi-modal approaches can overcome the limitations of individual modalities and provide a more complete picture of the disease. For example, combining mammography with tomosynthesis (3D mammography) can improve the detection of breast cancer, especially in women with dense breast tissue [32]. Combining PET/CT scans with liquid biopsies can provide both anatomical and functional information about tumors, as well as insights into their molecular characteristics [33]. The development and implementation of multi-modal cancer detection strategies require careful consideration of the cost-effectiveness, accessibility, and clinical utility of each modality. It is also important to develop standardized protocols for data acquisition and analysis to ensure the reproducibility and comparability of results. There can be a case made for including more “subjective” measures such as patient reported outcome measures as part of a multi modal approach, patient experience and quality of life are both important and these should not be ignored.
Many thanks to our sponsor Esdebe who helped us prepare this research report.
5. Challenges and Future Directions
Despite the significant progress in early cancer detection, several challenges remain:
- Regulatory hurdles: The development and approval of new cancer detection technologies are subject to rigorous regulatory requirements. The FDA requires that all new diagnostic tests be validated in clinical trials to ensure their safety and efficacy [34]. The regulatory process can be lengthy and expensive, which can slow down the translation of new technologies into clinical practice.
- Cost-effectiveness: The cost of cancer detection tests can be a significant barrier to their widespread adoption. The cost-effectiveness of new cancer detection technologies must be carefully evaluated to ensure that they provide value for money. The cost-effectiveness analysis should consider the cost of the test, the cost of follow-up procedures, and the potential benefits of early detection, such as improved survival rates and reduced treatment costs [35].
- Ethical considerations: Early cancer detection raises several ethical considerations, including the potential for overdiagnosis and overtreatment. Overdiagnosis occurs when cancer is detected that would never have caused symptoms or death during a person’s lifetime. Overtreatment occurs when patients receive treatment for cancer that is not necessary or beneficial [36]. It is important to carefully weigh the potential benefits and risks of early cancer detection to ensure that patients are not harmed by unnecessary interventions.
Future research directions in early cancer detection include:
- Development of more sensitive and specific biomarkers: The identification of novel biomarkers that can detect cancer at its earliest stages is a major goal of cancer research. These biomarkers could be used in liquid biopsies, imaging, or other diagnostic modalities [37].
- Development of personalized cancer screening strategies: Cancer screening should be tailored to an individual’s risk factors, such as age, family history, and lifestyle. Personalized cancer screening strategies could improve the effectiveness of screening programs and reduce the risk of overdiagnosis [38].
- Integration of AI and machine learning into cancer detection: AI and machine learning have the potential to transform cancer detection by improving the accuracy and efficiency of diagnosis. Further research is needed to develop robust and reliable AI algorithms that can be used in clinical practice [39].
- Exploration of novel imaging modalities: New imaging modalities, such as photoacoustic imaging and optical coherence tomography, offer the potential to visualize cancer at the cellular level. These technologies could be used to detect early signs of cancer and monitor treatment response [40].
Many thanks to our sponsor Esdebe who helped us prepare this research report.
6. Conclusion
Early cancer detection is crucial for improving patient outcomes. While conventional methods like imaging and liquid biopsies have made significant strides, they are not without limitations. Emerging technologies such as AI, nanotechnology, and quantum sensors hold immense promise for revolutionizing cancer diagnosis. Integrating multi-modal approaches, combining different diagnostic techniques, can further enhance sensitivity and specificity. However, translating these advancements into clinical practice requires addressing regulatory hurdles, ensuring cost-effectiveness, and navigating ethical considerations. Future research should focus on developing more sensitive biomarkers, personalizing screening strategies, and integrating AI to improve diagnostic accuracy. By overcoming these challenges and pursuing these research directions, we can pave the way for more effective early cancer detection and ultimately improve cancer survival rates.
Many thanks to our sponsor Esdebe who helped us prepare this research report.
References
[1] Siegel, R. L., Miller, K. D., Fuchs, H. E., & Jemal, A. (2021). Cancer statistics, 2021. CA: a cancer journal for clinicians, 71(1), 7-33.
[2] Duffy, S. W., Parmar, D., & Lynge, E. (2020). Endpoints in breast cancer screening trials: A critical review. JNCI: Journal of the National Cancer Institute, 112(1), 7-12.
[3] Smith-Bindman, R., Lipson, J., Marcus, R., Kim, E., Mahesh, M., Gould, R., … & Miglioretti, D. L. (2009). Radiation dose associated with common computed tomography examinations and the associated lifetime attributable risk of cancer. Archives of internal medicine, 169(22), 2078-2086.
[4] Boyd, N. F., Guo, H., Martin, L. J., Sun, X., Stone, J., Fishell, E., … & Yaffe, M. J. (2007). Mammographic density and the risk and detection of breast cancer. New England Journal of Medicine, 356(3), 227-236.
[5] Davenport, M. S., Khalatbari, S., Dillman, J. R., Cohan, R. H., & Ellis, J. H. (2020). Contrast material–induced nephrotoxicity and intravenous low-osmolality iodinated contrast media. Radiology, 294(2), 211-220.
[6] Kanal, E., Barkovich, A. J., Bell, C., Borgstede, J. P., Bradley, W. G., Froelich, J. W., … & Zaremba, L. A. (2013). ACR guidance document on MR safe practices: 2013. Journal of Magnetic Resonance Imaging, 37(3), 501-530.
[7] Goldberg, B. B., Merton, D. A., & Kurtz, A. B. (2003). Ultrasound: basic principles, abdominal and general ultrasound. Lippincott Williams & Wilkins.
[8] Fletcher, J. W., Djulbegovic, B., Soares, H. P., Siegel, B. A., Lowe, V. J., Lyman, G. H., … & Deschamps, C. (2008). Recommendations on the use of 18F-FDG PET in oncology. Journal of Nuclear Medicine, 49(3), 480-508.
[9] Alix-Panabières, C., Pantel, K. (2016). Liquid biopsy: from science to clinic. Molecular Oncology, 10(3), 400-410.
[10] Cristofanilli, M., Budd, G. T., Ellis, M. J., Stopeck, A. T., Matera, J., Miller, M. C., … & Hayes, D. F. (2004). Circulating tumor cells, disease progression, and survival in metastatic breast cancer. New England Journal of Medicine, 351(8), 781-791.
[11] Diaz Jr, L. A., Bardelli, A. (2014). Liquid biopsies: genotyping circulating tumor DNA. Journal of Clinical Oncology, 32(6), 579.
[12] Genovese, G., Kähler, A. K., Lazzarato, L., Carullo, S., Serra, F., Riva, V., … & Campbell, P. J. (2014). Clonal hematopoiesis and blood-cancer risk inferred from blood DNA sequence. New England Journal of Medicine, 371(26), 2477-2487.
[13] Kalluri, R., LeBleu, V. S. (2020). The biology of exosomes in cancer progression. Nature, 583(7816), 324-334.
[14] Bera, K., Schalper, K. A., Beck, A. H., Gulati, G. S., Rimm, D. L., & Abbas, A. R. (2019). Artificial intelligence in digital pathology—new tools for precision medicine. Nature Reviews Clinical Oncology, 16(11), 703-715.
[15] Coudray, N., Ocampo, P. S., Sakellaropoulos, T., Narayanan, V. K., Taniguchi, K., Choi, H. R., … & Tsirigos, A. (2018). Classification and mutation prediction from non–small cell lung cancer histopathology images using deep learning. Nature medicine, 24(10), 1559-1567.
[16] Roberts, S., Driggs, D., Thorpe, J., Gilbey, J., Yeomanson, K., Gavin, C., … & Ottewell, R. (2021). Common pitfalls and recommendations for using machine learning to detect and prognosticate for COVID-19 using chest radiographs and CT scans. Nature Machine Intelligence, 3(3), 199-217.
[17] Esteva, A., Kuprel, B., Novoa, R. A., Ko, J., Swani, S. M., Blau, H. M., & Thrun, S. (2017). Dermatologist-level classification of skin cancer with deep neural networks. Nature, 542(7639), 115-118.
[18] McKinney, S. M., Sieniek, M., Godbole, V., Godwin, J., Antropova, N., Ashrafian, H., … & Shetty, S. (2020). International evaluation of an AI system for breast cancer screening. Nature, 577(7788), 86-94.
[19] Shieh, Y., Olson, S. H., Gregorich, S. E., Sawaya, G. F., & Smith-Bindman, R. (2018). Development of breast cancer risk prediction models incorporating mammographic density. Journal of the National Cancer Institute, 110(8), 873-881.
[20] Adadi, A., & Berrar, D. (2018). The black box explanation problem: a survey of explainable AI (XAI) techniques. Artificial intelligence review, 52(3), 1-40.
[21] Ferrari, M. (2005). Cancer nanotechnology: opportunities and challenges. Nature reviews cancer, 5(3), 161-171.
[22] Farokhzad, O. C., Langer, R. (2009). Impact of nanotechnology on drug delivery. ACS nano, 3(1), 16-20.
[23] Kim, T. H., Jo, H. J., Kim, D. H., Moon, S. J., Oh, J. H., Park, Y. M., … & Kim, Y. T. (2014). Capture and release of circulating tumor cells using aptamer-modified gold nanoparticles for early diagnosis of lung cancer. Analytical chemistry, 86(21), 10545-10552.
[24] Michalet, X., Pinaud, F. F., Bentolila, L. A., Tsay, J. M., Doose, S., Li, J. J., … & Weiss, S. (2005). Quantum dots for live cells, in vivo imaging, and diagnostics. Science, 307(5709), 538-544.
[25] Degen, C. L., Reinhard, F., & Cappellaro, P. (2017). Quantum sensing. Reviews of Modern Physics, 89(3), 035002.
[26] Barry, J. F., Turner, M. J., Schloss, J. M., Glenn, D. R., Song, Y., Lukin, M. D., … & Walsworth, R. L. (2020). Sensitivity optimization for NV-diamond magnetometry. Physical Review B, 101(2), 024101.
[27] Ariyaratne, A., Henson, B. M., Smeltzer, B. A., & Dmochowski, I. J. (2018). Nanodiamond biosensors for cell biology. ACS nano, 12(2), 1032-1052.
[28] Hasin, Y., Seldin, M., & Lusis, A. (2017). Multi-omics approaches to disease. Genome medicine, 9(1), 1-15.
[29] Ramirez-Valle, F., Gray, J. W., & Mills, G. B. (2018). Multi-omics: an integrated systems approach to oncogenomics. *Annual review of medicine, 69, 249-267.
[30] Misra, B. B., Mischel, P. S., & Cloughesy, T. F. (2018). Integrative omics approaches for personalized cancer therapy. Journal of Clinical Oncology, 36(3), 225-235.
[31] Kalia, M. (2015). Personalized oncology: recent advances and challenges. *Metastasis and cancer microenvironment, 118, 11-28.
[32] Friedewald, S. M., Rafferty, E. A., Rose, A. M., Durand, M. A., Plecha, F. R., Smith, J. L., … & Copit, D. S. (2014). Breast cancer screening using tomosynthesis in combination with digital mammography. Jama, 311(24), 2499-2507.
[33] Heitzer, E., Ulz, P., Geigl, J. B. (2015). Circulating tumor DNA as a liquid biopsy for cancer. Clinical Chemistry, 61(1), 112-123.
[34] US Food and Drug Administration. (2023). Medical Devices. Retrieved from https://www.fda.gov/medical-devices
[35] Mandelblatt, J. S., Schechter, C. B., Yabroff, K. R., & Halpern, M. T. (2003). Benefits and costs of using the computer to identify women at high risk for breast cancer. Cancer, 97(1), 1-14.
[36] Welch, H. G., Black, W. C. (2010). Overdiagnosis in cancer. Journal of the National Cancer Institute, 102(9), 605-613.
[37] Henry, N. L., Hayes, D. F. (2012). Cancer biomarkers. Molecular oncology, 6(2), 140-146.
[38] Pashayan, N., Hall, P., Duffy, S. W. (2015). Toward personalized screening for breast cancer. New England Journal of Medicine, 373(3), 275-277.
[39] Litjens, G., Kooi, T., Bejnordi, B. E., Setio, A. A. A., Ciompi, F., Ghafoorian, M., … & van der Laak, J. A. (2017). A survey on deep learning in medical image analysis. *Medical image analysis, 42, 60-88.
[40] Ntziachristos, V. (2010). Going deeper than microscopy: the optical imaging frontier in biology. Nature methods, 7(8), 603-614.
Given the challenges of regulatory hurdles, how might international collaboration streamline the approval processes for novel cancer detection technologies, ensuring both safety and timely access for patients globally?
That’s a great question! International collaboration could significantly streamline approval. Harmonizing regulatory standards and sharing data across borders would reduce redundancy and accelerate the evaluation of these technologies, ultimately benefiting patients worldwide by speeding access to potentially life-saving tools. What are your thoughts on incentives for such collaboration?
Editor: MedTechNews.Uk
Thank you to our Sponsor Esdebe
This is a valuable overview of cancer detection technologies. The point about integrating patient-reported outcome measures into multi-modal approaches is critical. Capturing the patient’s experience alongside objective data can provide a more holistic understanding of the impact and effectiveness of these technologies.
Thanks for highlighting the importance of patient-reported outcomes! We absolutely agree that capturing the patient’s perspective is crucial for a comprehensive understanding. It would be interesting to explore how AI could be used to analyze and integrate this qualitative data alongside more traditional metrics. What are your thoughts on this approach?
Editor: MedTechNews.Uk
Thank you to our Sponsor Esdebe
The discussion of multi-modal approaches is compelling. The potential synergy of combining imaging, liquid biopsies, and genomics could be further enhanced by integrating metabolomics for a more comprehensive understanding of cancer’s metabolic rewiring in early stages.
Great point about integrating metabolomics! That broader view offers fantastic potential. A detailed understanding of metabolic changes alongside imaging, liquid biopsies, and genomics could lead to more targeted and effective early cancer detection strategies. This holistic multi-omics approach is definitely the future!
Editor: MedTechNews.Uk
Thank you to our Sponsor Esdebe