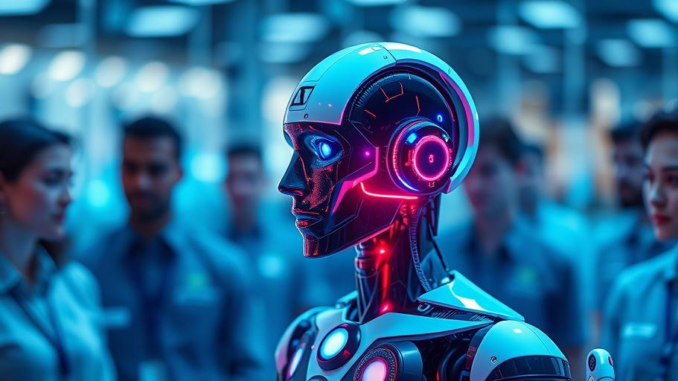
Abstract
Healthcare documentation, traditionally viewed as a repository of patient information, has evolved into a complex ecosystem influencing clinical decision-making, quality improvement, research, and regulatory compliance. This research report explores the evolving landscape of healthcare documentation, focusing on the critical aspects of interoperability, semantic standardization, and the enduring challenges to achieving meaningful use. We delve into the limitations of current documentation practices, examining the impact of unstructured data, the complexities of terminology mapping, and the persistent difficulties in integrating disparate systems. Furthermore, the report analyzes the role of emerging technologies, such as natural language processing (NLP) and artificial intelligence (AI), in transforming healthcare documentation from a static record into a dynamic and actionable resource. Finally, we discuss the implications for patient safety, data privacy, and the future of healthcare delivery in a documentation-rich environment.
Many thanks to our sponsor Esdebe who helped us prepare this research report.
1. Introduction
Healthcare documentation serves as the cornerstone of patient care, providing a comprehensive record of medical history, diagnoses, treatments, and outcomes. However, the transition from paper-based records to electronic health records (EHRs) has not fully realized its potential. While EHRs offer significant advantages in terms of accessibility and storage, challenges remain in achieving true interoperability, ensuring data quality, and maximizing the value of documented information for clinical and research purposes. The increasing volume of data generated by EHRs, coupled with the lack of standardized formats and terminologies, has created a complex and often fragmented documentation landscape. This complexity hinders the ability of healthcare providers to effectively access, interpret, and utilize patient information, potentially leading to errors, inefficiencies, and compromised patient safety. Moreover, the burden of documentation on clinicians has been cited as a major contributor to burnout and decreased job satisfaction. Therefore, a comprehensive understanding of the challenges and opportunities in healthcare documentation is crucial for improving the quality, safety, and efficiency of healthcare delivery.
Many thanks to our sponsor Esdebe who helped us prepare this research report.
2. Interoperability: A Persistent Challenge
Interoperability, the ability of different information systems to exchange and use data, is essential for effective healthcare documentation. However, despite significant investments in EHR technology, true interoperability remains elusive. Several factors contribute to this persistent challenge.
2.1 Technical Interoperability
Technical interoperability focuses on the ability of systems to exchange data, often relying on standardized protocols and formats. However, variations in implementation and the lack of universal adoption of standards hinder seamless data exchange. Different EHR vendors may use different protocols or proprietary formats, making it difficult for systems to communicate effectively. Even when standard protocols are used, inconsistencies in interpretation and implementation can lead to errors and data loss.
2.2 Semantic Interoperability
Semantic interoperability addresses the ability of systems to understand and interpret exchanged data in a consistent and meaningful way. This requires the use of standardized terminologies and coding systems, such as ICD-10, SNOMED CT, and LOINC. However, even with standardized terminologies, challenges remain in mapping different terminologies, resolving ambiguities, and ensuring that data is consistently coded across different systems. The lack of semantic interoperability can lead to misinterpretations, inconsistencies, and difficulties in aggregating data for analysis and reporting.
2.3 Organizational Interoperability
Organizational interoperability focuses on the policies, procedures, and governance structures that facilitate data sharing and collaboration across different healthcare organizations. This requires addressing issues such as data privacy, security, and trust. Different organizations may have different policies regarding data sharing, which can hinder the exchange of information. Concerns about data privacy and security can also limit the willingness of organizations to share data. Furthermore, the lack of trust between organizations can impede collaboration and data sharing efforts.
Many thanks to our sponsor Esdebe who helped us prepare this research report.
3. The Semantic Web and Healthcare Documentation
The Semantic Web offers a promising approach to enhancing semantic interoperability in healthcare documentation. By using ontologies and knowledge representation techniques, the Semantic Web can enable machines to understand and reason about the meaning of data. This can facilitate the integration of disparate data sources, improve the accuracy of clinical decision support systems, and enhance the efficiency of research and quality improvement efforts. Ontologies provide a formal representation of knowledge in a particular domain, defining the concepts, relationships, and axioms that govern the domain. By using ontologies to represent clinical knowledge, healthcare providers can ensure that data is interpreted consistently across different systems and organizations.
3.1 Application of Semantic Web Technologies
Semantic Web technologies, such as Resource Description Framework (RDF) and Web Ontology Language (OWL), are increasingly being used to develop healthcare applications. For example, RDF can be used to represent patient data in a standardized format, while OWL can be used to define ontologies for clinical domains. These technologies can facilitate the integration of data from different sources, such as EHRs, clinical databases, and research repositories.
3.2 Challenges in Semantic Web Implementation
Despite the potential benefits, implementing Semantic Web technologies in healthcare documentation faces several challenges. One challenge is the complexity of developing and maintaining ontologies. Ontologies require significant expertise in knowledge representation and domain knowledge. Another challenge is the need to integrate Semantic Web technologies with existing healthcare systems. This requires adapting existing systems to support RDF and OWL, which can be a complex and time-consuming process. Furthermore, the performance of Semantic Web applications can be a concern, especially when dealing with large volumes of data. Optimizing the performance of these applications requires careful design and implementation.
Many thanks to our sponsor Esdebe who helped us prepare this research report.
4. Natural Language Processing (NLP) and Unstructured Data
A significant portion of healthcare documentation consists of unstructured text, such as clinical notes, discharge summaries, and radiology reports. This unstructured data contains valuable information that is not easily accessible or analyzable using traditional methods. Natural language processing (NLP) can be used to extract structured information from unstructured text, enabling healthcare providers to better understand patient data and improve clinical decision-making. NLP techniques can be used to identify key concepts, relationships, and entities in unstructured text. For example, NLP can be used to identify diagnoses, medications, and procedures mentioned in clinical notes.
4.1 NLP Techniques for Healthcare
Various NLP techniques are used in healthcare, including named entity recognition, relation extraction, and sentiment analysis. Named entity recognition identifies and classifies entities in text, such as diseases, medications, and procedures. Relation extraction identifies relationships between entities, such as the relationship between a disease and a medication. Sentiment analysis identifies the sentiment expressed in text, such as positive, negative, or neutral. These techniques can be used to extract valuable information from unstructured text and improve the accuracy of clinical decision support systems.
4.2 Limitations of NLP
Despite its potential, NLP has limitations in healthcare. One limitation is the variability in clinical language. Clinicians may use different terms and abbreviations to describe the same condition. Another limitation is the presence of errors and inconsistencies in clinical text. NLP systems must be robust enough to handle these errors and inconsistencies. Furthermore, the performance of NLP systems can be affected by the quality and quantity of training data. Developing NLP systems that are accurate and reliable requires large amounts of high-quality training data.
Many thanks to our sponsor Esdebe who helped us prepare this research report.
5. The Impact of Documentation on Clinical Workflows and Clinician Burden
The increasing burden of documentation on clinicians has been a growing concern in recent years. Studies have shown that clinicians spend a significant portion of their time documenting patient information, often at the expense of direct patient care. This can lead to burnout, decreased job satisfaction, and potentially compromised patient safety. The complexity of EHR systems, the lack of standardized documentation templates, and the increasing regulatory requirements contribute to the documentation burden. Clinicians are often required to enter the same information multiple times in different parts of the EHR, which is time-consuming and inefficient. Furthermore, the lack of integration between different EHR systems can make it difficult for clinicians to access and utilize patient information from different sources.
5.1 Strategies to Reduce Clinician Burden
Several strategies can be used to reduce the documentation burden on clinicians. One strategy is to streamline documentation workflows. This can involve simplifying documentation templates, automating data entry, and integrating different EHR systems. Another strategy is to provide clinicians with better training on EHR systems. This can help clinicians to use the systems more efficiently and effectively. Furthermore, healthcare organizations can provide clinicians with administrative support to assist with documentation tasks. This can free up clinicians to focus on direct patient care.
5.2 The Role of AI in Reducing Burden
AI has the potential to significantly reduce the documentation burden on clinicians. AI-powered tools can automate many of the tasks currently performed by clinicians, such as transcribing notes, summarizing patient information, and identifying relevant clinical data. For example, AI can be used to automatically generate progress notes based on physician-patient conversations. AI can also be used to identify potential drug interactions and other clinical risks. By automating these tasks, AI can free up clinicians to focus on direct patient care and improve the efficiency of healthcare delivery.
Many thanks to our sponsor Esdebe who helped us prepare this research report.
6. Legal and Ethical Considerations
Healthcare documentation is subject to various legal and ethical considerations, including data privacy, security, and confidentiality. The Health Insurance Portability and Accountability Act (HIPAA) sets standards for the privacy and security of protected health information (PHI). Healthcare organizations must implement policies and procedures to protect PHI from unauthorized access, use, and disclosure. Violations of HIPAA can result in significant fines and penalties. Furthermore, healthcare organizations must comply with other laws and regulations related to data privacy and security, such as the General Data Protection Regulation (GDPR) in Europe. Ethical considerations also play a role in healthcare documentation. Clinicians have a responsibility to document patient information accurately and completely. They must also respect patient confidentiality and privacy. The use of EHRs and other electronic systems raises new ethical challenges, such as the potential for data breaches and the misuse of patient information.
6.1 Data Security and Privacy
Data security and privacy are paramount in healthcare documentation. Healthcare organizations must implement robust security measures to protect PHI from unauthorized access, use, and disclosure. These measures should include physical security, technical security, and administrative security. Physical security measures include controlling access to facilities and equipment. Technical security measures include using encryption, firewalls, and intrusion detection systems. Administrative security measures include implementing policies and procedures for data access, use, and disclosure. Furthermore, healthcare organizations must train their employees on data security and privacy best practices.
6.2 Consent and Patient Access
Patients have the right to access their medical records and to control the use and disclosure of their PHI. Healthcare organizations must provide patients with access to their medical records in a timely and convenient manner. They must also obtain patient consent before using or disclosing PHI for purposes other than treatment, payment, or healthcare operations. Patients have the right to request amendments to their medical records if they believe that the information is inaccurate or incomplete. Healthcare organizations must review and respond to patient requests for amendments in a timely manner.
Many thanks to our sponsor Esdebe who helped us prepare this research report.
7. Best Practices for Documentation Management
Effective documentation management is essential for ensuring data quality, patient safety, and regulatory compliance. Healthcare organizations should implement best practices for documentation management, including establishing clear policies and procedures, providing comprehensive training, and implementing quality assurance measures.
7.1 Standardized Templates and Workflows
Standardized templates and workflows can improve the consistency and accuracy of documentation. Healthcare organizations should develop standardized templates for common clinical documents, such as progress notes, discharge summaries, and referral letters. These templates should include all of the necessary information and should be designed to be easy to use. Healthcare organizations should also develop standardized workflows for documentation tasks, such as data entry, review, and approval. These workflows should ensure that documentation is completed in a timely and efficient manner.
7.2 Training and Education
Comprehensive training and education are essential for ensuring that clinicians are able to use EHR systems effectively and document patient information accurately. Healthcare organizations should provide clinicians with initial training on EHR systems and ongoing training on new features and updates. Training should cover all aspects of EHR use, including data entry, retrieval, and reporting. Healthcare organizations should also provide clinicians with training on documentation best practices, such as using standardized terminologies and avoiding abbreviations.
7.3 Quality Assurance and Auditing
Quality assurance and auditing are essential for ensuring that documentation is accurate and complete. Healthcare organizations should implement quality assurance measures to monitor the accuracy and completeness of documentation. These measures should include regular audits of medical records to identify errors and inconsistencies. Healthcare organizations should also provide feedback to clinicians on their documentation performance. This feedback should be used to improve documentation practices and reduce errors.
Many thanks to our sponsor Esdebe who helped us prepare this research report.
8. The Future of Healthcare Documentation
The future of healthcare documentation will be shaped by emerging technologies, such as AI, blockchain, and cloud computing. AI will play an increasingly important role in automating documentation tasks, improving the accuracy of clinical decision support systems, and enhancing the efficiency of healthcare delivery. Blockchain technology has the potential to improve data security and privacy by providing a secure and transparent platform for sharing patient information. Cloud computing can provide a scalable and cost-effective infrastructure for storing and managing healthcare data.
8.1 AI-Driven Documentation
AI will revolutionize healthcare documentation by automating many of the tasks currently performed by clinicians. AI-powered tools can transcribe notes, summarize patient information, and identify relevant clinical data. AI can also be used to generate progress notes based on physician-patient conversations. These tools can free up clinicians to focus on direct patient care and improve the efficiency of healthcare delivery.
8.2 Blockchain for Data Security
Blockchain technology can improve data security and privacy by providing a secure and transparent platform for sharing patient information. Blockchain uses cryptography to create a tamper-proof record of transactions. This makes it difficult for unauthorized users to access or modify patient data. Blockchain can also be used to track the provenance of data, ensuring that it is accurate and reliable.
8.3 Cloud-Based Solutions
Cloud computing can provide a scalable and cost-effective infrastructure for storing and managing healthcare data. Cloud-based solutions offer several advantages over traditional on-premise systems, including lower costs, greater scalability, and improved security. Cloud providers can also provide advanced security features, such as encryption and intrusion detection systems. However, healthcare organizations must carefully evaluate cloud providers to ensure that they meet their data privacy and security requirements.
Many thanks to our sponsor Esdebe who helped us prepare this research report.
9. Conclusion
Healthcare documentation is a critical component of patient care, but it faces significant challenges in terms of interoperability, data quality, and clinician burden. Addressing these challenges requires a multi-faceted approach that includes standardized terminologies, improved NLP techniques, streamlined workflows, and the adoption of emerging technologies. By embracing these strategies, healthcare organizations can transform healthcare documentation from a burden into a valuable asset that improves patient safety, enhances clinical decision-making, and supports research and quality improvement efforts. The integration of AI and Semantic web technologies promises to reshape how documentation is created, managed, and leveraged, ultimately paving the way for a more efficient and patient-centered healthcare system.
Many thanks to our sponsor Esdebe who helped us prepare this research report.
References
- American Medical Informatics Association. (n.d.). Interoperability. Retrieved from https://amia.org/
- HealthIT.gov. (n.d.). What is Interoperability? Retrieved from https://www.healthit.gov/
- HIPAA.gov. (n.d.). Summary of the HIPAA Security Rule. Retrieved from https://www.hhs.gov/hipaa/index.html
- Jiang, F., Jiang, Y., Xiao, Y., Dong, Y., Li, S., Zhang, H., Wang, Y., & Li, L. (2017). Artificial intelligence in healthcare: past, present and future. Stroke and vascular neurology, 2(4), 230-243.
- Liu, X., Chen, H., & Sun, J. (2019). Natural language processing in healthcare: recent advances and emerging trends. Journal of Biomedical Informatics, 99, 103244.
- Shortliffe, E. H., & Cimino, J. J. (2014). Biomedical informatics: computer applications in health care and biomedicine. Springer.
- Sittig, D. F., Singh, H., & Ash, J. S. (2010). Errors in electronic health records. Journal of the American Medical Informatics Association, 17(5), 481-489.
- Verheij, G. G., Peek, N., & Hurskainen, H. (2020). Semantic interoperability in healthcare: A review. International Journal of Medical Informatics, 139, 104144.
The discussion on AI’s potential to reduce clinician burden is compelling. Beyond automating tasks, could AI also assist in real-time clinical decision support by analyzing documented data and suggesting best practice guidelines or flagging potential risks, further optimizing workflows?
That’s a great point! AI’s role in real-time clinical decision support could significantly enhance best practice adherence and risk mitigation. Imagine AI proactively flagging potential medication interactions based on a patient’s complete history, leading to safer and more effective treatment plans. How might we ensure that AI-driven suggestions remain a supportive tool rather than a replacement for clinical judgment?
Editor: MedTechNews.Uk
Thank you to our Sponsor Esdebe
The report highlights the critical need for semantic interoperability. Standardizing terminology and coding systems across platforms is essential, and exploring blockchain’s potential for secure data sharing could significantly advance this effort.
Thanks for highlighting semantic interoperability, it’s definitely a key area! I agree that blockchain offers exciting possibilities for secure data sharing in healthcare. How do you think we can best address the regulatory hurdles associated with implementing blockchain in this sector to ensure patient privacy and data governance?
Editor: MedTechNews.Uk
Thank you to our Sponsor Esdebe