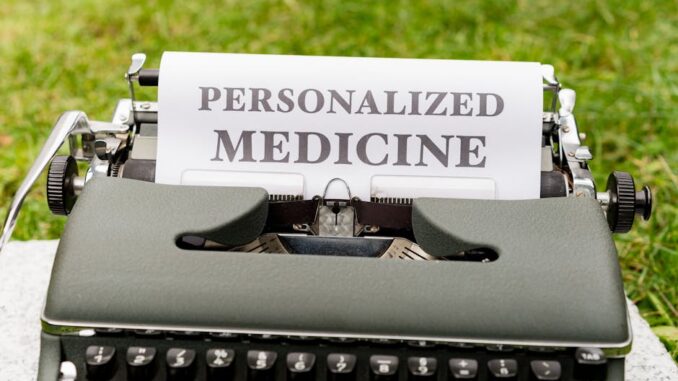
Abstract
Pharmacology, the study of drug action, is undergoing a significant transformation driven by advancements in artificial intelligence (AI), pharmacogenomics, and systems pharmacology. This research report explores the multifaceted impact of these converging fields on drug discovery, personalized medicine, and pharmacology education. We delve into how AI is revolutionizing drug target identification, lead compound optimization, and clinical trial design, while pharmacogenomics is enabling personalized treatment strategies based on individual genetic profiles. Furthermore, we examine the application of systems pharmacology in understanding complex drug-body interactions at a holistic level. Finally, we address the crucial role of AI-powered educational tools in equipping future healthcare professionals with the necessary pharmacological knowledge and skills in this rapidly evolving landscape. This report aims to provide a comprehensive overview of the current state and future directions of pharmacology, highlighting the potential for improved therapeutic outcomes and patient safety.
Many thanks to our sponsor Esdebe who helped us prepare this research report.
1. Introduction
Pharmacology stands at the intersection of chemistry, biology, and medicine, playing a crucial role in understanding how drugs interact with living organisms to produce therapeutic effects. Traditionally, pharmacology has relied on empirical methods, often involving time-consuming and resource-intensive laboratory experiments and clinical trials. However, the advent of high-throughput screening, genomics, proteomics, and other omics technologies has generated vast amounts of data, creating new opportunities for accelerating drug discovery and development. The interpretation and analysis of such large datasets requires sophisticated computational tools and approaches, leading to the integration of artificial intelligence (AI) and machine learning (ML) into pharmacological research. This integration has the potential to drastically improve efficacy, safety and the speed to market of novel therapeutics.
Moreover, the growing recognition of inter-individual variability in drug response has fueled the rise of pharmacogenomics, which investigates the influence of genetic variations on drug metabolism and efficacy. By identifying genetic biomarkers that predict drug response, pharmacogenomics enables the development of personalized treatment strategies, minimizing adverse drug reactions and maximizing therapeutic benefits. This moves medicine from a “one size fits all” approach, to one that delivers care catered to individual patients.
Systems pharmacology, a related field, takes a more holistic approach by considering the complex interactions between drugs, biological systems, and environmental factors. By integrating data from various sources, including genomics, proteomics, metabolomics, and clinical data, systems pharmacology aims to develop predictive models of drug action at the system level. These models can be used to identify novel drug targets, predict drug efficacy and toxicity, and optimize drug dosing regimens. These techniques, alongside the rapidly expanding field of AI will revolutionize all areas of pharmacology.
Finally, the increasing complexity of pharmacological knowledge necessitates innovative educational approaches to train future healthcare professionals. The incorporation of AI-powered tools in pharmacology education offers the potential to personalize learning, enhance understanding of drug mechanisms and interactions, and improve medication safety knowledge. This ensures that future healthcare practitioners are well equipped to utilize this evolving science to its fullest potential.
Many thanks to our sponsor Esdebe who helped us prepare this research report.
2. Artificial Intelligence in Drug Discovery
AI is transforming drug discovery across multiple stages, from target identification to lead optimization and clinical trial design. Traditional target identification methods often rely on serendipitous discoveries or hypothesis-driven research, which can be inefficient and time-consuming. AI algorithms, on the other hand, can analyze vast amounts of biological data, including genomic, proteomic, and transcriptomic data, to identify novel drug targets that are implicated in disease pathogenesis. For example, machine learning models can be trained to predict the likelihood of a protein being a druggable target based on its structural features, expression patterns, and interactions with other proteins. These models can then prioritize potential targets for further validation.
Once a promising target has been identified, AI can accelerate the process of lead compound discovery. Virtual screening techniques, powered by AI algorithms, can screen millions of compounds in silico to identify those that are likely to bind to the target and modulate its activity. These techniques can significantly reduce the number of compounds that need to be synthesized and tested experimentally, saving time and resources. Furthermore, AI can be used to optimize lead compounds by predicting their pharmacokinetic and pharmacodynamic properties. Machine learning models can be trained on large datasets of compound structures and activity data to predict how a compound will be absorbed, distributed, metabolized, and excreted by the body (ADME properties), as well as its potency and selectivity for the target.
AI is also playing an increasing role in clinical trial design and analysis. Machine learning models can be used to identify patient subgroups that are most likely to respond to a particular drug, allowing for more targeted and efficient clinical trials. These models can also be used to predict adverse drug reactions and optimize dosing regimens. Furthermore, AI can automate the analysis of clinical trial data, reducing the time and cost associated with traditional statistical methods. For example, natural language processing (NLP) techniques can be used to extract relevant information from electronic health records and clinical trial reports, streamlining the process of data collection and analysis.
Despite the great promise of AI in drug discovery, there are also challenges that need to be addressed. One major challenge is the lack of high-quality data. AI algorithms are only as good as the data they are trained on, so it is essential to ensure that the data is accurate, complete, and representative of the population of interest. Another challenge is the lack of interpretability of some AI models. Complex machine learning models, such as deep neural networks, can be difficult to understand, making it challenging to validate their predictions and identify potential biases. As AI is further integrated into the drug discovery process, it is important to be aware of these limitations and to use AI in conjunction with traditional methods to ensure the safety and efficacy of new drugs.
Many thanks to our sponsor Esdebe who helped us prepare this research report.
3. Pharmacogenomics and Personalized Medicine
Pharmacogenomics is the study of how genes affect a person’s response to drugs. It aims to develop personalized treatment strategies based on an individual’s genetic makeup. Genetic variations can influence drug metabolism, drug transport, and drug target interactions, leading to differences in drug efficacy and toxicity among individuals. By identifying genetic biomarkers that predict drug response, pharmacogenomics can help clinicians select the right drug and the right dose for each patient, minimizing adverse drug reactions and maximizing therapeutic benefits.
One of the most well-established applications of pharmacogenomics is in the field of oncology. For example, patients with certain mutations in the EGFR gene are more likely to respond to EGFR inhibitors, such as gefitinib and erlotinib. Similarly, patients with certain mutations in the BRAF gene are more likely to respond to BRAF inhibitors, such as vemurafenib and dabrafenib. By testing patients for these mutations before initiating treatment, clinicians can select the most effective therapy and avoid unnecessary side effects.
Pharmacogenomics is also being applied to other areas of medicine, such as cardiology, psychiatry, and infectious diseases. For example, variations in the CYP2C19 gene can affect the metabolism of clopidogrel, an antiplatelet drug used to prevent blood clots. Patients with certain CYP2C19 variants may not metabolize clopidogrel effectively, increasing their risk of stroke or heart attack. By testing patients for CYP2C19 variants before prescribing clopidogrel, clinicians can identify those who may benefit from an alternative antiplatelet therapy.
Despite the great potential of pharmacogenomics, there are also challenges that need to be addressed. One major challenge is the cost of genetic testing. While the cost of sequencing has decreased dramatically in recent years, it is still relatively expensive to perform pharmacogenomic testing on a large scale. Another challenge is the lack of clinical guidelines for the use of pharmacogenomic testing in many areas of medicine. While there are some well-established pharmacogenomic tests, such as those for EGFR and BRAF in oncology, there is a need for more research and clinical trials to validate the use of pharmacogenomic testing in other areas of medicine. In addition, ethical considerations surrounding genetic privacy and the potential for genetic discrimination need to be carefully addressed. The responsible implementation of pharmacogenomics requires a collaborative effort among researchers, clinicians, policymakers, and patients.
Many thanks to our sponsor Esdebe who helped us prepare this research report.
4. Systems Pharmacology: A Holistic Approach
Systems pharmacology represents a paradigm shift from traditional pharmacology by considering the complex interactions between drugs, biological systems, and environmental factors. It integrates data from various sources, including genomics, proteomics, metabolomics, and clinical data, to develop predictive models of drug action at the system level. These models can be used to identify novel drug targets, predict drug efficacy and toxicity, and optimize drug dosing regimens.
One of the key concepts in systems pharmacology is the idea that drugs can have multiple effects on the body, not just a single effect on a single target. This is because drugs can interact with multiple proteins, enzymes, and signaling pathways, leading to a cascade of downstream effects. Systems pharmacology aims to capture these complex interactions and predict the overall effect of a drug on the body. For example, a systems pharmacology model could be used to predict the effect of a drug on blood pressure, heart rate, and kidney function, taking into account the patient’s individual genetic background, medical history, and lifestyle factors.
Systems pharmacology also emphasizes the importance of considering the context in which a drug is administered. The effect of a drug can vary depending on the patient’s age, sex, weight, and other factors. Furthermore, the effect of a drug can be influenced by environmental factors, such as diet, exercise, and exposure to toxins. Systems pharmacology aims to capture these contextual factors and predict how they will influence drug response. This becomes incredibly important when considering the effects of polypharmacy. The effect of multiple drugs being administered, and their interaction can only truly be modelled using systems pharmacology.
Systems pharmacology models can be used to identify novel drug targets by identifying key nodes in biological networks that are disrupted in disease. These models can also be used to predict drug efficacy and toxicity by simulating the effect of a drug on the body. Furthermore, systems pharmacology models can be used to optimize drug dosing regimens by predicting the optimal dose for each patient based on their individual characteristics.
The challenges facing systems pharmacology are similar to those facing AI and pharmacogenomics. These include the need for high quality data, and the potential for biased results if data is not representative of the broader population. These challenges are being overcome however, and systems pharmacology is becoming a crucial tool for enhancing the efficacy and safety of novel therapeutics.
Many thanks to our sponsor Esdebe who helped us prepare this research report.
5. AI-Powered Tools in Pharmacology Education
The integration of AI-powered tools in pharmacology education offers the potential to revolutionize the way future healthcare professionals learn about drugs and their effects. Traditional pharmacology education often relies on memorization of drug names, mechanisms of action, and side effects, which can be challenging and ineffective. AI-powered tools can personalize learning, enhance understanding of drug mechanisms and interactions, and improve medication safety knowledge. The current state of pharmacology education requires significant reform, and these techniques may lead to that revolution.
One promising application of AI in pharmacology education is the use of virtual patient encounters. Virtual patient encounters allow students to practice their clinical skills in a safe and controlled environment. Students can interview virtual patients, order diagnostic tests, and prescribe medications, receiving feedback on their decisions from an AI-powered tutor. These virtual patient encounters can help students develop critical thinking skills and improve their understanding of how to apply pharmacological knowledge in clinical practice.
Another application of AI in pharmacology education is the use of AI-powered tutoring systems. These systems can provide students with personalized feedback on their understanding of pharmacological concepts. The AI-powered tutor can assess the student’s knowledge and identify areas where they need additional help. The tutor can then provide customized learning materials and practice questions to help the student improve their understanding. Furthermore, AI-powered tutoring systems can track student progress and provide feedback to instructors, allowing them to tailor their teaching to the needs of their students. This is more efficient than one to one learning, providing a better student experience.
AI can also be used to create interactive simulations of drug mechanisms of action. These simulations can help students visualize how drugs interact with biological targets and how these interactions lead to therapeutic effects. By manipulating the variables in the simulation, students can explore the effects of different drugs and doses on the body. This can help students develop a deeper understanding of pharmacological principles and improve their ability to predict drug responses. Further, AI-powered tools can enhance students’ understanding of drug interactions. These tools can simulate the effects of combining different drugs, allowing students to explore the potential for synergistic or antagonistic interactions. This can help students develop a better understanding of the risks and benefits of polypharmacy.
The integration of AI-powered tools in pharmacology education has the potential to improve medication safety knowledge among future healthcare professionals. Medication errors are a leading cause of preventable harm in healthcare, and many medication errors are due to a lack of knowledge about drugs and their interactions. By providing students with personalized learning, interactive simulations, and virtual patient encounters, AI-powered tools can help them develop a deeper understanding of pharmacological principles and improve their ability to prescribe medications safely and effectively. This will have profound implications for the safety and efficacy of therapeutics in the future.
Many thanks to our sponsor Esdebe who helped us prepare this research report.
6. Future Directions and Challenges
The fields of AI, pharmacogenomics, and systems pharmacology are rapidly evolving, and their integration into pharmacology has the potential to revolutionize drug discovery, personalized medicine, and pharmacology education. However, there are also challenges that need to be addressed to fully realize the potential of these technologies.
One major challenge is the need for more high-quality data. AI algorithms, pharmacogenomic analyses, and systems pharmacology models are only as good as the data they are trained on, so it is essential to ensure that the data is accurate, complete, and representative of the population of interest. This requires the development of standardized data collection methods and the creation of large, publicly available datasets. Data sharing and collaboration between researchers, clinicians, and industry partners are also crucial for accelerating progress in these fields.
Another challenge is the need for more sophisticated computational tools and algorithms. Many of the current AI algorithms and systems pharmacology models are complex and difficult to interpret. There is a need for more transparent and interpretable models that can be used to understand the underlying mechanisms of drug action and predict drug responses. Furthermore, there is a need for more user-friendly software tools that can be used by researchers and clinicians to analyze data and make informed decisions.
The translation of research findings into clinical practice is also a major challenge. While there are many promising applications of AI, pharmacogenomics, and systems pharmacology, few of these applications have been widely adopted in clinical practice. This is due in part to the lack of clinical guidelines and reimbursement policies for these technologies. There is a need for more clinical trials to validate the use of these technologies and to develop evidence-based guidelines for their implementation. Furthermore, there is a need for education and training programs to equip clinicians with the knowledge and skills to use these technologies effectively.
Finally, ethical considerations surrounding the use of AI, pharmacogenomics, and systems pharmacology need to be carefully addressed. These technologies raise important ethical questions about genetic privacy, data security, and the potential for bias and discrimination. It is essential to develop ethical guidelines and regulations to ensure that these technologies are used responsibly and for the benefit of all patients.
Many thanks to our sponsor Esdebe who helped us prepare this research report.
7. Conclusion
The integration of AI, pharmacogenomics, and systems pharmacology is transforming the landscape of pharmacology, offering unprecedented opportunities for drug discovery, personalized medicine, and enhanced education. AI is accelerating drug target identification, lead optimization, and clinical trial design. Pharmacogenomics is enabling personalized treatment strategies based on individual genetic profiles. Systems pharmacology is providing a holistic understanding of drug-body interactions. AI-powered educational tools are equipping future healthcare professionals with the necessary pharmacological knowledge and skills.
While significant challenges remain, the potential benefits of these technologies are immense. By addressing these challenges and fostering collaboration among researchers, clinicians, industry partners, and policymakers, we can unlock the full potential of AI, pharmacogenomics, and systems pharmacology to improve therapeutic outcomes and patient safety. The future of pharmacology is undoubtedly intertwined with these transformative technologies, promising a new era of precision medicine and personalized healthcare.
Many thanks to our sponsor Esdebe who helped us prepare this research report.
References
- Vamathevan, J., Clark, D., Czodrowski, P., Eswaran, K., Giangreco, M. R., Szalai, B., … & Bender, A. (2019). Applications of machine learning in drug discovery and development. Nature Reviews Drug Discovery, 18(6), 463-477.
- Relling, M. V., & Evans, W. E. (2015). Pharmacogenomics in the clinic. Nature, 526(7573), 343-350.
- Berger, A. C., Iyengar, R., & Yadav, B. (2019). Systems pharmacology. Nature Biotechnology, 37(8), 846-855.
- Zheng, X., Patel, N. P., Johnson, D. L., Williamson, P. R., Balakrishnan, R., & Arnold, R. J. (2023). Artificial intelligence in clinical pharmacology education: A systematic review. Journal of Clinical Pharmacology, 63(11), 1267-1279.
- Chan, I. S., Bentzen, S. M., Schildt, B. M., Sutcliffe, P., Clark, S. J., & Altman, D. G. (2008). Sample size for binary outcome trials with non-adherence. Statistics in Medicine, 27(11), 1988-2001.
- Hood, L., & Friend, S. H. (2011). Predictive, personalized, preventive, and participatory (P4) cancer medicine. Nature Reviews Clinical Oncology, 8(3), 184-187.
- Hamburg, M. A., & Collins, F. S. (2010). The path to personalized medicine. New England Journal of Medicine, 363(4), 301-304.
- Lesko, L. J., Atkinson, A. J., Jr. (2001). Translational pharmacodynamics/pharmacokinetics of anticancer drugs. Clinical Pharmacology & Therapeutics, 69(6), 365-368.
- Davies, J. A., & Delany, C. (2023). AI-Enabled Drug Discovery: Opportunities and Challenges. Trends in Pharmacological Sciences, 44(5), 271-273.
Given the increasing complexity of pharmacological knowledge, how might AI-powered educational tools adapt to teach nuanced drug interactions and personalized medicine approaches effectively?
That’s a great point! AI could really shine by creating personalized learning paths that adapt to each student’s understanding of drug interactions. Imagine simulations where students can explore the impact of genetic variations on drug response in real-time! It’s an exciting area to watch develop.
Editor: MedTechNews.Uk
Thank you to our Sponsor Esdebe
AI revolutionizing drug discovery? Fascinating! But shouldn’t we be equally concerned about the potential for AI to perpetuate existing biases in drug development, leading to therapies that disproportionately benefit certain populations? Just a thought.
That’s a really important point! Addressing potential biases in AI-driven drug discovery is crucial. We should explore ways to ensure that AI algorithms are trained on diverse datasets and that the resulting therapies are accessible and beneficial to all populations. It’s a complex issue that warrants careful consideration and proactive solutions.
Editor: MedTechNews.Uk
Thank you to our Sponsor Esdebe