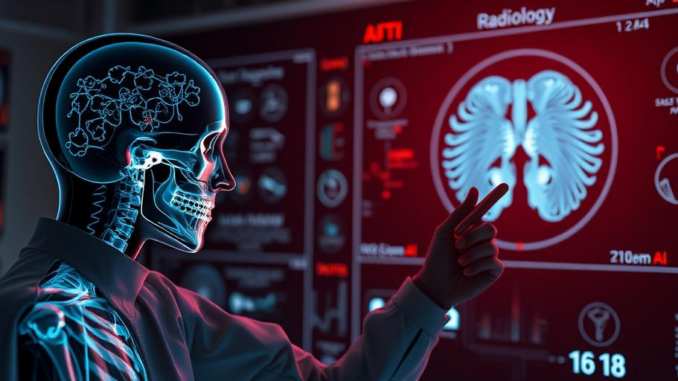
Abstract
The integration of Artificial Intelligence (AI) into radiology is no longer a futuristic concept but a rapidly evolving reality. This research report provides a comprehensive analysis of the evolving role of the radiologist in the age of AI, moving beyond simple comparisons of AI’s diagnostic accuracy to encompass a broader perspective on how AI fundamentally alters the radiologist’s workflow, responsibilities, and skill set. We explore the current applications of AI in image acquisition, processing, interpretation, and reporting, critically evaluating its performance relative to human radiologists across various modalities and disease states. Furthermore, the report delves into the potential future trends, including personalized medicine, proactive diagnostics, and the integration of multimodal data, while also addressing the significant challenges posed by data bias, algorithmic transparency, regulatory hurdles, and the ethical considerations surrounding AI deployment. Ultimately, we argue that the future of radiology lies not in AI replacing radiologists, but in a collaborative synergy where AI augments human expertise, allowing radiologists to focus on higher-level tasks, personalized patient care, and the advancement of the field as a whole.
Many thanks to our sponsor Esdebe who helped us prepare this research report.
1. Introduction
Radiology, at its core, is the art and science of interpreting medical images to diagnose and monitor disease. For over a century, radiologists have served as crucial gatekeepers of patient health, leveraging their expertise to extract meaningful information from complex visual data. However, the sheer volume and complexity of medical imaging data have increased exponentially in recent years, driven by advancements in imaging technologies such as computed tomography (CT), magnetic resonance imaging (MRI), and positron emission tomography (PET). This data deluge, coupled with increasing demands for faster turnaround times and improved diagnostic accuracy, has placed significant strain on radiologists, leading to potential for burnout and diagnostic errors [1].
The emergence of artificial intelligence, particularly deep learning, offers a promising solution to alleviate these challenges. AI algorithms have demonstrated remarkable capabilities in image recognition and pattern analysis, enabling them to detect subtle anomalies and quantify disease burden with unprecedented speed and accuracy. While early discussions often focused on the potential for AI to replace radiologists entirely, a more nuanced perspective has emerged, emphasizing the role of AI as a powerful tool to augment and enhance human expertise. This report adopts this perspective, examining how AI is reshaping the radiologist’s role, skill set, and responsibilities in the 21st century. We will explore the current applications of AI in radiology, critically assess its performance against human radiologists, and discuss the future trends and challenges associated with its widespread adoption.
Many thanks to our sponsor Esdebe who helped us prepare this research report.
2. Current Applications of AI in Radiology
AI is currently being deployed across a wide spectrum of radiological applications, ranging from image acquisition and processing to interpretation and reporting. Each stage presents unique opportunities for AI to improve efficiency, accuracy, and patient outcomes.
2.1. Image Acquisition and Processing:
AI is playing an increasingly important role in optimizing image acquisition protocols and enhancing image quality. For example, AI-powered algorithms can automatically adjust scan parameters based on patient anatomy and clinical indication, reducing radiation dose and improving image contrast [2]. In MRI, AI-based reconstruction techniques are used to accelerate scan times and reduce image artifacts, leading to improved patient comfort and throughput [3]. Furthermore, AI can be used for image denoising, artifact reduction, and image enhancement, resulting in clearer and more interpretable images.
2.2. Image Interpretation and Diagnosis:
This is perhaps the most widely publicized application of AI in radiology. Numerous studies have demonstrated the ability of AI algorithms to detect and classify various diseases with accuracy comparable to, and in some cases exceeding, that of human radiologists. Examples include:
- Lung Nodule Detection: AI algorithms can automatically detect and quantify pulmonary nodules on CT scans, potentially leading to earlier diagnosis of lung cancer [4].
- Breast Cancer Screening: AI-based solutions are being used to assist radiologists in screening mammograms, improving sensitivity and reducing false positive rates [5].
- Stroke Detection: AI can rapidly identify signs of acute stroke on CT scans, enabling faster treatment and improved outcomes [6].
- Fracture Detection: AI algorithms can accurately detect fractures on radiographs, reducing the risk of missed diagnoses [7].
- Cardiovascular Imaging: AI is being used to quantify cardiac chamber volumes, assess coronary artery stenosis, and detect myocardial infarction [8].
These are just a few examples of the many applications of AI in image interpretation. However, it’s important to note that the performance of AI algorithms can vary depending on the specific disease, imaging modality, and patient population. Furthermore, the “black box” nature of some AI algorithms raises concerns about interpretability and explainability, making it difficult for radiologists to understand how the algorithm arrived at its decision. This is a critical area of ongoing research.
2.3. Reporting and Workflow Optimization:
AI can also be used to streamline the reporting process and optimize radiologist workflow. Natural language processing (NLP) algorithms can automatically extract key findings from medical images and generate preliminary reports, saving radiologists time and effort [9]. AI-powered workflow management systems can prioritize cases based on urgency and complexity, ensuring that the most critical cases are addressed promptly [10]. Furthermore, AI can be used to identify potential discrepancies between image findings and clinical information, alerting radiologists to potential errors.
Many thanks to our sponsor Esdebe who helped us prepare this research report.
3. Comparing AI Performance with Human Radiologists
While AI has demonstrated impressive capabilities in various radiological tasks, it’s crucial to critically evaluate its performance relative to human radiologists. Several studies have compared the diagnostic accuracy of AI algorithms with that of experienced radiologists, yielding mixed results.
In some cases, AI has outperformed human radiologists, particularly in tasks involving the detection of subtle anomalies or the quantification of large datasets. For example, studies have shown that AI algorithms can detect pulmonary nodules with higher sensitivity and specificity than radiologists [4]. Similarly, AI has demonstrated superior performance in quantifying cardiac chamber volumes and assessing coronary artery stenosis [8].
However, in other cases, human radiologists have maintained an edge, particularly in tasks requiring contextual understanding, complex reasoning, and the integration of clinical information. Radiologists are trained to consider the patient’s medical history, physical examination findings, and laboratory results when interpreting medical images, allowing them to make more informed and accurate diagnoses. AI algorithms, on the other hand, typically rely solely on image data, which can limit their ability to handle complex cases.
Furthermore, the performance of AI algorithms can be highly dependent on the quality and representativeness of the training data. If the training data is biased or incomplete, the algorithm may perform poorly on unseen data. This is a significant concern, as many AI algorithms are trained on data from specific patient populations or institutions, which may not be representative of the general population.
It’s also important to consider the concept of “algorithmic bias.” AI algorithms can inadvertently perpetuate or amplify existing biases in the data they are trained on, leading to disparities in diagnostic accuracy across different demographic groups. For example, an AI algorithm trained on mammograms from predominantly white women may perform poorly on mammograms from women of other ethnicities [11].
Ultimately, the optimal approach is likely a collaborative one, where AI augments human expertise. AI can be used to pre-screen images, identify potential abnormalities, and quantify disease burden, freeing up radiologists to focus on more complex cases, integrate clinical information, and provide personalized patient care.
Many thanks to our sponsor Esdebe who helped us prepare this research report.
4. Future Trends and Potential Challenges
The future of radiology is inextricably linked to the continued advancement of AI. Several emerging trends hold the potential to revolutionize the field, but they also present significant challenges that must be addressed.
4.1. Personalized Medicine:
AI is poised to play a central role in the development of personalized medicine approaches in radiology. By integrating image data with genomic information, clinical data, and lifestyle factors, AI algorithms can predict an individual’s risk of disease, tailor treatment strategies, and monitor treatment response. For example, AI can be used to predict which patients are most likely to benefit from specific cancer therapies based on their tumor characteristics and genomic profile [12].
4.2. Proactive Diagnostics:
Traditionally, radiology has been primarily reactive, focusing on diagnosing disease after symptoms have already appeared. However, AI can enable a more proactive approach to diagnostics, allowing for the early detection of disease before it progresses to an advanced stage. For example, AI can be used to identify individuals at high risk of developing Alzheimer’s disease based on subtle changes in brain structure and function on MRI scans [13].
4.3. Integration of Multimodal Data:
The future of radiology will likely involve the integration of data from multiple sources, including imaging, genomics, proteomics, and electronic health records. AI algorithms can be used to analyze and integrate these diverse datasets, providing a more comprehensive and holistic view of the patient’s health. This integrated approach will enable more accurate diagnoses, personalized treatment plans, and improved patient outcomes.
4.4. Challenges to Overcome:
Despite the immense potential of AI in radiology, several challenges must be addressed to ensure its safe and effective implementation:
- Data Bias: As mentioned earlier, data bias is a significant concern. AI algorithms are only as good as the data they are trained on, and biased data can lead to inaccurate and unfair diagnoses. Efforts must be made to ensure that training data is representative of the general population and that algorithms are rigorously tested for bias.
- Algorithmic Transparency: The “black box” nature of some AI algorithms raises concerns about interpretability and explainability. Radiologists need to understand how the algorithm arrived at its decision in order to trust its output. Research is needed to develop more transparent and explainable AI algorithms.
- Regulatory Hurdles: The regulatory landscape for AI in radiology is still evolving. Clear guidelines and standards are needed to ensure the safety and efficacy of AI-based diagnostic tools. Regulatory agencies must work with industry and academia to develop appropriate regulations that promote innovation while protecting patient safety.
- Ethical Considerations: The widespread adoption of AI in radiology raises several ethical considerations, including data privacy, algorithmic bias, and the potential for job displacement. These ethical issues must be addressed proactively to ensure that AI is used responsibly and ethically.
- Cost and Accessibility: The development and implementation of AI-based radiology solutions can be expensive, potentially limiting access to advanced diagnostic tools for underserved populations. Efforts must be made to reduce the cost of AI and ensure that it is accessible to all patients, regardless of their socioeconomic status.
- Integration into existing workflows: Integrating new AI solutions into the already complex hospital workflows can be challenging. This includes issues of interoperability with existing PACS systems, EMRs, and other clinical systems. Seamless integration is crucial for efficient and effective use of AI tools.
Many thanks to our sponsor Esdebe who helped us prepare this research report.
5. The Evolving Role of the Radiologist
The integration of AI into radiology is not about replacing radiologists, but about augmenting their expertise and allowing them to focus on higher-level tasks. The role of the radiologist is evolving from a primarily image-reading function to a more consultative and patient-centered role.
In the future, radiologists will likely spend less time on routine image interpretation and more time on complex cases, multidisciplinary collaboration, and personalized patient care. They will serve as experts in the integration of imaging data with other clinical information, providing valuable insights to clinicians and patients.
Radiologists will also play a critical role in the development and validation of AI algorithms. They will work with data scientists and engineers to ensure that AI algorithms are accurate, reliable, and unbiased. They will also be responsible for monitoring the performance of AI algorithms in clinical practice and identifying potential problems.
Ultimately, the future of radiology lies in a collaborative synergy between humans and AI. AI will augment human expertise, allowing radiologists to focus on higher-level tasks, personalized patient care, and the advancement of the field as a whole. This collaboration will require a shift in training and education, with radiologists needing to develop new skills in data science, AI, and personalized medicine.
Many thanks to our sponsor Esdebe who helped us prepare this research report.
6. Conclusion
The integration of AI into radiology is transforming the field at an unprecedented pace. AI is already being used to improve image acquisition, enhance image quality, assist in image interpretation, and optimize workflow. While AI has demonstrated impressive capabilities in various radiological tasks, it’s crucial to critically evaluate its performance relative to human radiologists and address the challenges posed by data bias, algorithmic transparency, regulatory hurdles, and ethical considerations.
The future of radiology lies not in AI replacing radiologists, but in a collaborative synergy where AI augments human expertise. The role of the radiologist is evolving from a primarily image-reading function to a more consultative and patient-centered role. Radiologists will play a critical role in the development, validation, and implementation of AI algorithms, ensuring that they are used safely, effectively, and ethically. By embracing the opportunities presented by AI, radiologists can enhance patient care, improve diagnostic accuracy, and advance the field of radiology as a whole.
Many thanks to our sponsor Esdebe who helped us prepare this research report.
References
[1] Berlin, L. (2017). Radiology malpractice: what to learn from 199 cases. American Journal of Roentgenology, 209(2), 273-281.
[2] Zhang, Y., et al. (2020). Deep learning-based automated tube current modulation for CT imaging. Medical Physics, 47(10), 5100-5111.
[3] Knoll, F., et al. (2020). Deep learning for accelerated MRI: A review. Journal of Magnetic Resonance Imaging, 53(1), 33-56.
[4] Ardila, D., et al. (2019). End-to-end lung cancer screening with three-dimensional deep learning on low-dose chest computed tomography. Nature Medicine, 25(6), 954-961.
[5] McKinney, S. M., et al. (2020). International evaluation of an AI system for breast cancer screening. Nature, 577(7788), 89-94.
[6] Chilamkurti, N. K., et al. (2018). Deep learning and medical image analysis for stroke diagnosis: A survey. Computers in Biology and Medicine, 95, 198-215.
[7] Lindsey, R., et al. (2018). Deep learning for fracture detection using convolutional neural networks. Skeletal Radiology, 47(6), 709-717.
[8] Attia, Z. I., et al. (2019). An artificial intelligence–enabled ECG algorithm for the identification of patients with atrial fibrillation during normal sinus rhythm: Rationale and design of the screening to prevent strokes in atrial fibrillation (STOP-AF) trial. American Heart Journal, 216, 58-66.
[9] Irvin, J., et al. (2019). CheXpert: A large chest radiograph dataset and competition for automated disease detection. arXiv preprint arXiv:1901.07031.
[10] Prevedello, L. M., et al. (2017). Radiology workflow management: A systematic review. Journal of the American College of Radiology, 14(6), 721-732.
[11] Zech, J. R., et al. (2018). Variable generalization performance of a deep learning model to detect pneumonia from chest radiographs: A retrospective study. PLoS Medicine, 15(11), e1002683.
[12] Aerts, H. J. W. L., et al. (2014). Decoding tumour phenotype by noninvasive image analysis. Nature Communications, 5(1), 1-9.
[13] Risacher, S. L., et al. (2010). Baseline MRI predictors of Alzheimer’s disease: The ADNI cohort. Brain, 133(11), 3285-3298.
The discussion around algorithmic bias is particularly relevant. What steps can be taken to ensure AI training data in radiology reflects diverse demographics, thus mitigating potential disparities in diagnostic accuracy across different patient populations?