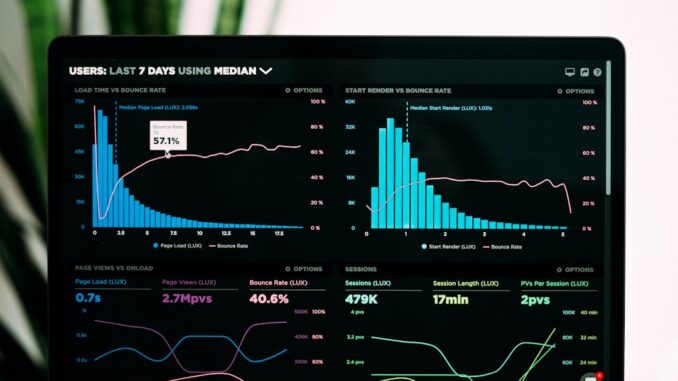
Summary
This article explores how predictive analytics can revolutionize SDOH screening by leveraging existing EHR data and machine learning. It discusses a recent study where a predictive model outperformed traditional questionnaires in anticipating patient needs. Finally, it addresses the importance of mitigating bias in these models to ensure equitable healthcare access.
** Main Story**
Social determinants of health, or SDOH, things like not having enough food, unstable housing, and trouble getting around, they really hit hard when it comes to how healthy we are. You know, it’s much more than just seeing a doctor. Historically, healthcare providers have used questionnaires to try and figure out what social needs people have. But let’s be honest, those questionnaires? They can be a pain, and sometimes they don’t even give you the full picture, do they?
Enter predictive analytics, powered by the magic of AI and machine learning (ML). This is where things get interesting. By looking at the data we already have in electronic health records (EHRs), these models can spot patterns and even predict what health-related social needs patients might face in the future.
A Look at How Well Predictive Analytics Works
There was this recent study in PLOS One that really highlights how much potential predictive analytics has for SDOH screening. Researchers built an ML model using EHR data from patients who had already filled out an SDOH survey. The model used natural language processing (NLP) to dig into clinical notes and other data points, looking for signs of social needs. And guess what? When they compared it to the usual screening questionnaire, the ML model was better at predicting patients’ needs for social services over the next month. Thirty days, and it made a difference! That means predictive analytics could help healthcare organizations get ahead of the curve, anticipating SDOH needs and stepping in earlier with the right support.
Funny thing, though: the study also found that adding basic stuff like age and gender to the old questionnaire made it more accurate. Goes to show, even simple data can make a difference.
Tackling Bias: A Must-Do
Okay, so the findings are pretty exciting, right? But there’s a big ‘but’ here: we have to think about bias in these models. Honestly, if we don’t, we risk making things worse. The study actually showed that both the ML model and the questionnaire did better at predicting SDOH needs for White, non-Hispanic patients. Why? Well, it probably comes down to unequal access to healthcare and biases in the data itself. Things like having insurance, where you live, if there’s a language barrier – all that can affect what data ends up in EHRs, and that can skew the predictions.
So, what do we do? We need to find ways to reduce bias in these models so they help everyone, no matter their background. One idea is to use area deprivation index scores. These give us context about neighborhoods where patients live, which can help level the playing field when it comes to access to resources. I remember, we had a project where we didn’t address the bias early enough, the results were completely skewed and we had to start all over again.
AI: More Than Just SDOH Screening
The use of AI in healthcare? It’s huge, way beyond just SDOH screening. Think of it: AI-powered tools are changing everything from helping doctors make decisions to analyzing medical images. AI can help diagnose diseases earlier and more accurately, create personalized treatment plans, and even take care of some of the administrative stuff, freeing up time for patient care.
For example, AI systems are being used to detect sepsis way before you’d see clinical symptoms, so doctors can step in quickly and potentially save lives. And in medical imaging, AI algorithms can spot tiny signs of diseases like breast cancer that a human radiologist might miss. That’s a game-changer, isn’t it?
AI-Driven Healthcare: A Bright Future
Honestly, the potential of AI to improve healthcare is enormous. By using predictive analytics and other AI technologies, healthcare systems can be more proactive, efficient, and, most importantly, focused on the patient. In SDOH, AI can help close health gaps and make sure everyone has the resources they need to thrive.
That said, we have to be responsible about how we develop and use these tools, tackling bias and prioritizing fairness. The future of healthcare, in my opinion, lies in carefully combining AI, human knowledge, and a dedication to making healthcare fair for everyone. It’s a journey, but it’s one worth taking.
The study’s findings on the ML model outperforming traditional questionnaires are compelling. It raises questions about the potential for integrating diverse data sources, like community resource directories, to further enhance the accuracy and scope of predictive analytics in addressing SDOH.