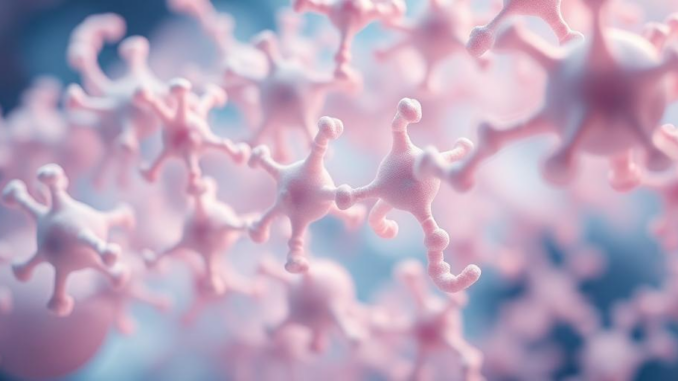
Abstract
The proteome, encompassing the entire complement of proteins expressed by a cell, tissue, or organism at a given time, represents a dynamic and complex entity that is central to biological function. Unlike the relatively static genome, the proteome is highly responsive to environmental cues and developmental stage, reflecting the intricate regulation of gene expression and post-translational modifications (PTMs). This research report provides a comprehensive overview of the current state of proteomic research, delving into cutting-edge technologies, persistent challenges, and the burgeoning applications that extend far beyond specific disease areas. We explore advancements in mass spectrometry-based proteomics, including quantitative strategies and approaches for characterizing PTMs and protein interactions. Furthermore, we discuss the computational and bioinformatic tools essential for handling and interpreting the massive datasets generated by proteomic experiments. Finally, we examine the expanding role of proteomics in systems biology, drug discovery, personalized medicine, and understanding fundamental biological processes, emphasizing the importance of integrating proteomic data with other omics datasets for a holistic view of cellular function.
Many thanks to our sponsor Esdebe who helped us prepare this research report.
1. Introduction
The field of proteomics has emerged as a cornerstone of modern biological research, offering a powerful lens through which to examine the complex world of proteins. Proteins are the workhorses of the cell, performing a vast array of functions, including catalysis, signaling, structural support, and transport. The proteome, therefore, provides a more direct representation of cellular phenotype than the genome or transcriptome. While genomics reveals the potential to produce proteins, and transcriptomics quantifies the levels of mRNA transcripts, proteomics directly measures the abundance, modifications, and interactions of proteins, providing critical insights into cellular state and function. The proteome’s dynamic nature necessitates continuous analysis to capture snapshots of cellular processes in response to various stimuli. These measurements provide a window into health and disease at a resolution far exceeding the capabilities of the human eye or traditional methods of biochemical analysis.
However, the complexity of the proteome presents formidable challenges. The sheer number of proteins, coupled with the vast array of PTMs and protein isoforms, makes comprehensive analysis a daunting task. Furthermore, the dynamic range of protein abundance, spanning several orders of magnitude, requires highly sensitive and accurate analytical techniques. As we continue to push the boundaries of proteomic technology, it becomes crucial to address these challenges in order to fully unlock the potential of the proteome for understanding and manipulating biological systems.
Many thanks to our sponsor Esdebe who helped us prepare this research report.
2. Advancements in Mass Spectrometry-Based Proteomics
Mass spectrometry (MS) has become the predominant technology for proteomic analysis, owing to its high sensitivity, accuracy, and throughput. MS-based proteomics typically involves digesting proteins into peptides using enzymes like trypsin, followed by separation of the peptides using liquid chromatography (LC) and analysis by MS. The MS instrument measures the mass-to-charge ratio (m/z) of the peptides, which can then be used to identify the proteins from which they originated. Over the past two decades, significant advancements in MS technology have revolutionized the field, enabling increasingly comprehensive and quantitative proteomic analyses.
2.1 Quantitative Proteomics Strategies
Quantifying protein abundance is essential for understanding changes in protein expression under different conditions or in response to stimuli. Several quantitative proteomics strategies have been developed, which can be broadly classified into two categories: label-free quantification and labeled quantification.
-
Label-Free Quantification (LFQ): LFQ relies on comparing the intensity or spectral counts of peptides across different samples without the use of isotopic labels. The advantage of LFQ is that it is relatively simple and cost-effective. However, it can be less accurate and reproducible than labeled methods, particularly for complex samples. Popular LFQ approaches include intensity-based quantification, which correlates peptide peak intensities with protein abundance, and spectral counting, which counts the number of spectra identified for each protein.
-
Labeled Quantification: Labeled quantification involves incorporating stable isotopes into peptides, either metabolically (e.g., SILAC) or chemically (e.g., iTRAQ, TMT). These labels introduce mass differences between peptides from different samples, allowing for accurate and precise quantification. Stable Isotope Labeling by Amino acids in Cell culture (SILAC) is a popular method which can incorporate heavy isotopes into proteins when cells are grown in specific media containing the labelled amino acids. Isobaric tags for relative and absolute quantification (iTRAQ) and tandem mass tags (TMT) are chemical labeling methods that allow for multiplexed quantification of up to 18 samples simultaneously. Labeled quantification offers higher accuracy and precision than LFQ but requires additional sample preparation steps and can be more expensive.
2.2 Characterizing Post-Translational Modifications
PTMs play a crucial role in regulating protein function, localization, and interactions. Many proteins are subject to a multitude of PTMs. Analysis of modified peptides is especially challenging as modification can change the physical and chemical properties of the resulting peptide which impacts its ionization efficiency. Identifying and quantifying PTMs is therefore essential for a complete understanding of the proteome. MS-based proteomics has emerged as a powerful tool for characterizing PTMs, including phosphorylation, glycosylation, ubiquitination, and acetylation.
-
Enrichment Strategies: Due to the low stoichiometry of many PTMs, enrichment strategies are often required to increase the abundance of modified peptides prior to MS analysis. For example, phosphopeptides can be enriched using immobilized metal affinity chromatography (IMAC) or titanium dioxide (TiO2) chromatography. Glycopeptides can be enriched using lectin affinity chromatography or hydrophilic interaction liquid chromatography (HILIC).
-
PTM-Specific Mass Spectrometry: Specialized MS techniques, such as electron-transfer/higher-energy collision dissociation (EThcD), can be used to improve the identification and localization of PTMs. EThcD fragmentation provides complementary information to traditional collision-induced dissociation (CID), allowing for more confident assignment of PTM sites. These techniques can be used to not only identify the presence of a modification but also to pinpoint its exact location on the peptide sequence.
2.3 Advances in Protein Interaction Analysis
Proteins rarely function in isolation; they typically interact with other proteins to form complexes and networks that carry out cellular processes. Identifying protein-protein interactions (PPIs) is therefore critical for understanding cellular function. MS-based proteomics has become a major tool for studying PPIs.
-
Affinity Purification-Mass Spectrometry (AP-MS): AP-MS involves using an antibody or other affinity reagent to pull down a target protein and its interacting partners from a cell lysate. The co-purified proteins are then identified by MS. AP-MS is a powerful method for identifying direct and indirect protein interactions.
-
Cross-linking Mass Spectrometry (XL-MS): XL-MS involves using chemical cross-linkers to covalently link interacting proteins. The cross-linked proteins are then digested, and the cross-linked peptides are identified by MS. XL-MS provides structural information about protein complexes and can be used to map interaction interfaces. Many different types of cross linkers are available, including those which are cleavable by chemical or enzymatic methods that can simplify the analysis procedure.
-
Proximity Ligation Assay coupled with Mass Spectrometry (PLA-MS): PLA-MS is a relatively new method that combines the proximity ligation assay (PLA) with MS. PLA uses antibodies conjugated to DNA oligonucleotides that hybridize only when the target proteins are in close proximity. The hybridized DNA oligonucleotides are then amplified, and the amplified DNA is used to identify the interacting proteins by MS. PLA-MS is a highly sensitive method for detecting protein interactions in situ.
Many thanks to our sponsor Esdebe who helped us prepare this research report.
3. Computational and Bioinformatic Challenges
The advances in MS technology have led to an explosion of proteomic data. Managing, processing, and interpreting these massive datasets present significant computational and bioinformatic challenges. These can range from relatively simple database queries to complex machine learning models.
3.1 Data Processing and Analysis
The raw data generated by MS instruments must be processed to identify peptides and proteins. This process typically involves several steps, including peak picking, database searching, and statistical validation.
-
Database Searching: Database searching involves comparing the MS/MS spectra of unknown peptides against a database of protein sequences to identify the best matching peptide. Search engines such as Mascot, Sequest, and Andromeda are commonly used for this purpose. The choice of database search engine and search parameters can significantly impact the results.
-
Statistical Validation: Statistical validation is crucial to ensure the accuracy of protein identifications. This involves estimating the false discovery rate (FDR) and filtering out peptide-spectrum matches (PSMs) that are likely to be incorrect. Target-decoy approach is a common method for FDR estimation.
3.2 Data Integration and Interpretation
Proteomic data is most informative when integrated with other omics datasets, such as genomic, transcriptomic, and metabolomic data. This integration allows for a more holistic understanding of cellular function and regulation.
-
Pathway Analysis: Pathway analysis involves mapping proteins and their interactions onto known biological pathways to identify enriched pathways and regulatory networks. Tools such as KEGG, Reactome, and Gene Set Enrichment Analysis (GSEA) are commonly used for pathway analysis.
-
Network Analysis: Network analysis involves constructing protein-protein interaction networks and analyzing their topological properties to identify key regulatory proteins and modules. Tools such as Cytoscape and STRING are commonly used for network analysis.
3.3 Machine Learning and Artificial Intelligence
Machine learning and artificial intelligence are increasingly being used to analyze and interpret proteomic data. These methods can be used to predict protein function, identify disease biomarkers, and personalize treatment strategies. Machine learning algorithms can also automate tasks such as data processing and peak picking.
-
Biomarker Discovery: Machine learning algorithms can be trained to identify proteins that are differentially expressed in different disease states. These proteins can then be used as biomarkers for disease diagnosis, prognosis, and treatment monitoring. However, careful validation and consideration of bias is essential when developing such algorithms.
-
Drug Target Identification: Machine learning algorithms can be used to predict protein targets for drug development. These algorithms can integrate proteomic data with other omics datasets to identify proteins that are essential for disease progression and are therefore good targets for drug intervention.
Many thanks to our sponsor Esdebe who helped us prepare this research report.
4. Applications of Proteomics
Proteomics has found widespread applications in various fields, including medicine, biotechnology, and agriculture. Some of the key applications are outlined below.
4.1 Cancer Research
Cancer is a complex disease characterized by uncontrolled cell growth and proliferation. Proteomics has emerged as a powerful tool for understanding the molecular mechanisms underlying cancer development and progression.
-
Biomarker Discovery: Proteomics can be used to identify biomarkers for cancer diagnosis, prognosis, and treatment response. For example, proteomics has been used to identify biomarkers for early detection of ovarian cancer and to predict response to chemotherapy in breast cancer.
-
Drug Target Identification: Proteomics can be used to identify novel drug targets for cancer therapy. For example, proteomics has been used to identify proteins that are essential for cancer cell survival and proliferation, which can then be targeted by new drugs.
4.2 Drug Discovery and Development
Proteomics plays a crucial role in drug discovery and development by identifying drug targets, understanding drug mechanisms of action, and predicting drug toxicity.
-
Target Validation: Proteomics can be used to validate drug targets identified by other methods, such as genomics and transcriptomics. For example, proteomics can be used to confirm that a protein target is expressed in the relevant tissue and that it is modulated by the drug.
-
Mechanism of Action Studies: Proteomics can be used to understand the mechanism of action of drugs by identifying proteins that are modulated by the drug. This information can be used to optimize drug design and to identify potential side effects.
4.3 Personalized Medicine
Personalized medicine aims to tailor medical treatment to the individual patient based on their unique genetic and molecular profile. Proteomics plays a key role in personalized medicine by providing information about the patient’s protein expression profile.
-
Treatment Stratification: Proteomics can be used to stratify patients into different treatment groups based on their protein expression profile. This allows for more targeted and effective treatment strategies.
-
Drug Response Prediction: Proteomics can be used to predict a patient’s response to a particular drug based on their protein expression profile. This information can be used to select the most effective drug for each patient and to avoid prescribing drugs that are unlikely to be effective.
4.4 Systems Biology
Systems biology aims to understand biological systems as a whole by integrating data from different omics levels, including genomics, transcriptomics, proteomics, and metabolomics. Proteomics is an essential component of systems biology, providing information about the protein expression profile and protein interactions.
-
Network Modeling: Proteomic data can be used to construct network models of biological systems. These models can be used to simulate cellular processes and to predict the effects of perturbations, such as drug treatment or genetic mutations.
-
Dynamic Modeling: Proteomic data can be used to develop dynamic models of biological systems. These models can capture the dynamic behavior of cellular processes over time and can be used to understand how cells respond to stimuli.
Many thanks to our sponsor Esdebe who helped us prepare this research report.
5. Challenges and Future Directions
Despite the significant advancements in proteomic technology, several challenges remain. One major challenge is the complexity of the proteome, which makes it difficult to achieve comprehensive proteome coverage. Another challenge is the dynamic range of protein abundance, which requires highly sensitive and accurate analytical techniques. Additionally, the data generated by proteomic experiments is often complex and requires sophisticated bioinformatics tools for analysis and interpretation.
5.1 Addressing the Complexity of the Proteome
To address the complexity of the proteome, researchers are developing new methods for sample preparation, fractionation, and MS analysis. These methods aim to increase proteome coverage and to improve the sensitivity and accuracy of protein identification and quantification. In particular, efforts are being made to improve the analysis of low-abundance proteins and PTMs.
5.2 Improving the Dynamic Range of Protein Quantification
To improve the dynamic range of protein quantification, researchers are developing new MS techniques that can measure the abundance of both high-abundance and low-abundance proteins with high accuracy. These techniques often involve the use of internal standards and normalization procedures to correct for variations in sample preparation and MS analysis.
5.3 Enhancing Bioinformatics Tools
To enhance bioinformatics tools for proteomic data analysis, researchers are developing new algorithms and software packages that can handle the massive datasets generated by proteomic experiments. These tools often incorporate machine learning and artificial intelligence techniques to improve the accuracy and efficiency of data analysis.
5.4 Multi-Omics Integration
The future of proteomics lies in its integration with other omics technologies. By combining proteomic data with genomic, transcriptomic, and metabolomic data, researchers can gain a more holistic understanding of biological systems. This integration will require the development of new bioinformatics tools and analytical approaches that can handle the complexity of multi-omics data. It also requires careful experimental design to ensure that different omics datasets are compatible and can be integrated effectively. For example, synchronizing the timepoints of sampling across different omics experiments is critical.
5.5 Expanding Applications
As proteomic technologies continue to improve, their applications will expand to new areas. Proteomics is expected to play an increasingly important role in personalized medicine, drug discovery, and systems biology. In addition, proteomics is being applied to new areas, such as environmental monitoring and food safety. Furthermore, the development of new proteomic technologies, such as single-cell proteomics, will open up new avenues for research.
Many thanks to our sponsor Esdebe who helped us prepare this research report.
6. Conclusion
Proteomics has emerged as a powerful tool for understanding the complexity of biological systems. The continued development of new technologies and bioinformatics tools is enabling researchers to gain unprecedented insights into the proteome and its role in health and disease. By integrating proteomic data with other omics datasets, we can achieve a more holistic understanding of cellular function and regulation. The future of proteomics is bright, with the potential to revolutionize personalized medicine, drug discovery, and our understanding of fundamental biological processes.
Many thanks to our sponsor Esdebe who helped us prepare this research report.
References
- Aebersold, R., & Mann, M. (2003). Mass spectrometry-based proteomics. Nature, 422(7005), 198-207.
- Cox, J., & Mann, M. (2011). MaxQuant enables high peptide identification rates, individualized p.p.b.-range mass accuracies and proteome-wide protein quantification. Nature biotechnology, 26(12), 1367-1372.
- Gygi, S. P., Rist, B., Gerber, S. A., Turecek, F., Gelb, M. H., & Aebersold, R. (1999). Quantitative analysis of complex protein mixtures using isotope-coded affinity tags. Nature biotechnology, 17(10), 994-999.
- Ong, S. E., Blagoev, B., Kratchmarova, I., Kristensen, D. B., Steen, H., Pandey, A., & Mann, M. (2002). Stable isotope labeling by amino acids in cell culture, SILAC, as a simple and accurate approach to expression proteomics. Molecular & cellular proteomics, 1(5), 376-386.
- Paulo, J. A., & Gygi, S. P. (2017). Advances in mass spectrometry-based proteomics for biology and medicine. Current Opinion in Biotechnology, 47, 77-87.
- Sinitcyn, P., Hamzeiy, H., Gessulat, S., & Mann, M. (2016). False discovery rate estimation for proteomic cross-linking analysis with xQuest. Nature methods, 13(3), 237-240.
- Venkatesan, K., Rual, J. F., Vazquez, A., Stelzl, U., Lemmens, I., Hirozane-Kishikawa, T., … & Vidal, M. (2009). An empirical framework for binary protein–protein interactions. Nature methods, 6(1), 83-90.
- Wilhelm, M., Schlegl, J., Hahne, H., Gholami, A. M., Lieberenz, M., Savitski, M. M., … & Kuster, B. (2014). Mass spectrometry–based proteomics of human cells and tissues. Nature, 509(7502), 582-587.
- Zubarev, R. A., Kruger, N. A., Fridriksson, E. K., Stahl, D. C., Schroder, B. M., Mann, M., & Kelleher, N. L. (2000). Electron capture dissociation for structural analysis of singly charged biomolecules. Journal of the American Chemical Society, 122(43), 10792-10799.
The discussion of multi-omics integration highlights a significant trend. How do you see the challenges of standardizing data formats and analytical pipelines across different omics platforms being addressed to facilitate more seamless integration?
That’s a great point! Standardizing data formats and analytical pipelines is crucial. I think community-driven initiatives, like developing common data exchange formats and shared analysis workflows, will play a key role. Investment in open-source tools and platforms will also greatly facilitate seamless integration across different omics platforms.
Editor: MedTechNews.Uk
Thank you to our Sponsor Esdebe